Discover the seemingly infinite number of AI use cases for business. As the adoption of generative AI continues to expand, new applications for the technology are revealed almost every day. Real estate, procurement, insurance, finance, and healthcare represent a mere fraction of the fields integrating AI into business processes. Let’s explore some of the top artificial intelligence use cases for generating business value below.

Generative AI Use Cases for Real Estate
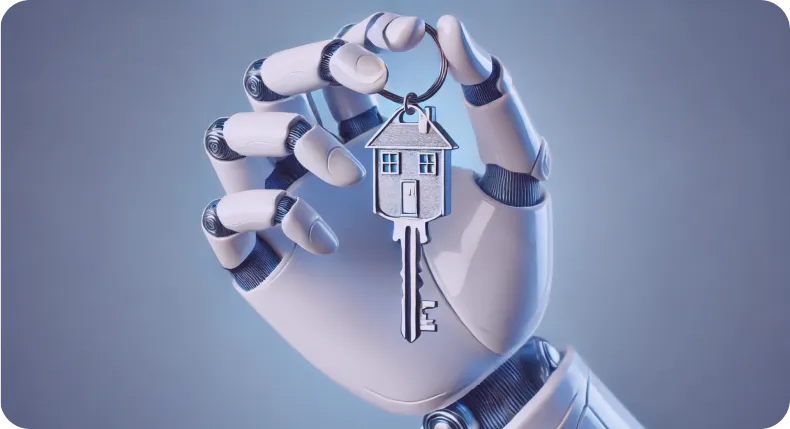
24/7 Customer Service
Conversational AI agents in real estate facilitate continuous customer engagement with 24/7 availability. These advanced chatbots process natural language queries from potential property buyers, leveraging deep learning models to interpret and respond to listing-specific inquiries and automate appointment scheduling. AI agents execute multi-step dialogue flows, addressing frequently asked questions through knowledge base integration, while implementing intelligent escalation protocols to human agents for complex scenarios. This architecture ensures uninterrupted service delivery and optimizes the customer journey during peak demand periods.
Custom Home-Buying Experience
Generative AI-powered customer service agents provide round-the-clock personalized assistance to prospective homebuyers. These advanced systems leverage natural language processing (NLP) to interpret user preferences, dynamically respond to property listing inquiries, automate viewing schedules, and disseminate geospecific neighborhood data. Chatbots employ machine learning (ML) algorithms to continuously refine their interaction models, increasing user engagement and information accuracy.
Faster Transactions
Natural language processing models and ML algorithms streamline real estate transactions by automating document authentication and contract lifecycle management. Predictive analytics powered by artificial intelligence extracts actionable insights from large datasets, enabling data-driven decision-making. Conversational AI agents leveraging deep learning techniques deliver personalized, context-aware customer support through natural language interfaces.
Automate Property Analysis
Machine learning algorithms process expansive real estate datasets to execute comprehensive property analyses. These algorithms incorporate complex variables including geospatial information, amenity proximity, and market trend indicators to generate precise valuations and investment intelligence.
By leveraging artificial neural networks to scrutinize historical market data, the system identifies high-potential investment opportunities within the real estate sector. This data-driven strategy enables stakeholders to make empirically supported decisions based on predictive modeling and pattern-recognition techniques.
Enhanced Data Processing
AI analyzes and parses through real estate documentation, performing automated information extraction and structured data organization. This process minimizes manual data entry requirements, mitigates error rates, and improves record fidelity.
Cognitive computing systems leverage NLP and computer vision techniques to automate relevant data extraction from mortgage instruments. These AI-powered systems optimize workflow efficiency and augment process automation in loan origination and servicing.
Generative AI Use Cases for Procurement

Inventory Management and Reordering
Generative AI virtual assistants employ predictive analytics algorithms to forecast inventory requirements, leveraging historical data sets to perform supply chain optimization. These systems utilize ML models to perform time-series data analysis, identifying patterns and trends in stock depletion rates.
Upon detecting inventory levels approaching predefined thresholds, the AI autonomously initiates procurement protocols, generating purchase orders with quantities determined by statistical demand-forecasting models. An AI-powered inventory management system maintains optimal stock levels while minimizing carrying costs and mitigating stockout risks. AI continuously refines its predictive models through reinforcement learning, adapting to shifting consumption patterns and enhancing operational efficiency.
Sourcing Products and Services
AI chatbots execute complex procurement workflows, leveraging natural language processing and decision-tree algorithms to navigate users through sourcing processes aligned with predefined organizational parameters and policies.
When a procurement specialist initiates a sourcing task, the AI system employs a conversational interface to elicit specific requirements through targeted query sequences. The chatbot then utilizes these inputs to query internal databases and external APIs, applying multi-criteria decision analysis to generate supplier recommendations that optimize for budget constraints and quality metrics.
Concurrently, the system executes automated price comparison routines across a pre-vetted vendor network, aggregating real-time pricing data. This AI-powered approach streamlines the sourcing process, eliminating manual catalog searches and vendor communications while maintaining adherence to corporate procurement guidelines.
Enterprise Knowledge Search
Gen AI technologies efficiently process all company documents, extracting and structuring data with high fidelity. This automation minimizes manual data entry, mitigates transcription errors, and enhances data integrity in record management systems.
ML models implement cognitive computing solutions for the procurement industry, autonomously extracting salient information from existing documentation. These systems optimize workflow processes and elevate operational efficiency through intelligent document processing and automated data extraction pipelines.
Price Tracking and Market Analysis
AI continuously monitors market dynamics, competitor pricing strategies, and supplier behavior patterns in real-time, generating actionable insights to optimize purchase decisions and negotiation tactics. Predictive models, leveraging historical datasets and current market indicators, forecast potential price fluctuations for specific commodities and materials.
These AI-powered forecasts enable procurement teams to implement data-driven purchasing strategies and execute more effective price negotiations with suppliers. The system's continuous learning capabilities allow it to adapt to changing market conditions, providing up-to-date intelligence for strategic sourcing decisions.
Generative AI Use Cases for Insurance
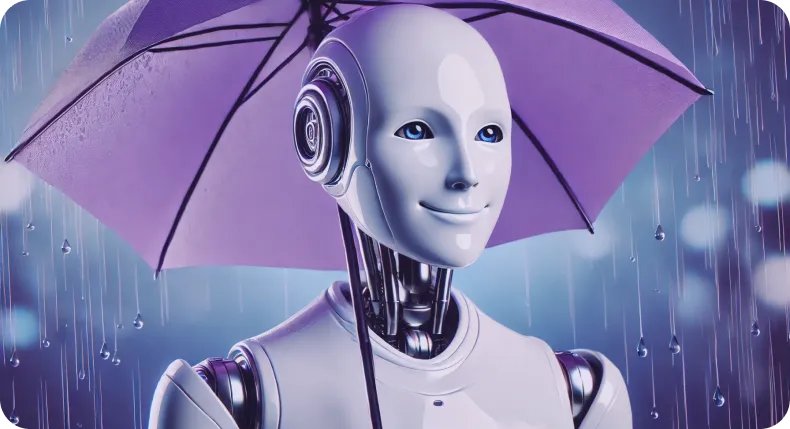
Custom Policy Management
Generative AI chatbots provide continuous policyholder support at all hours, facilitating real-time policy management, document retrieval, and information updates. These AI systems leverage natural language understanding algorithms to parse user queries and execute multi-step processes.
If a policyholder initiates pre-travel coverage verification, a chatbot accesses secure databases to retrieve policy-specific information. It then employs semantic analysis to interpret and respond to coverage-related inquiries, delineating inclusions and exclusions based on the policy's terms.
The chatbot also utilizes decision trees to determine query complexity, automatically escalating to human agents when predefined thresholds are exceeded. This AI-powered system optimizes information accessibility and improves user experience through rapid, accurate response generation.
Adaptive Customer Service
The latest generative AI chatbots employ predictive modeling algorithms to anticipate customer requirements, leveraging natural language processing to address high-frequency inquiries and generate personalized recommendations based on policy specifics and historical interaction data.
These AI tools use proactive notification protocols, utilizing time-based triggers to alert users of impending policy expirations. AI autonomously executes comparative quote analyses, dynamically adjusting coverage options based on user-specific parameters.
Simultaneously, the AI chatbot deploys a conversational interface to guide users through renewal, utilizing decision trees and knowledge graph traversal to provide context-aware responses. This application optimizes customer engagement, streamlines the renewal process, and promotes policy customization through data-driven insights.
Automated Risk Assessment and Underwriting
Gen AI assimilates and analyzes multidimensional datasets, including customer demographics and claims histories, to generate high-fidelity risk profiles for insurance products. These algorithms employ predictive modeling techniques to process automotive telematics data, meteorological information, and vehicle maintenance records.
By leveraging these data streams, the system forecasts accident probabilities for auto insurance applicants with increased accuracy. This data-driven approach facilitates precise risk stratification and generates tailored premium quotations based on individual risk factors and behavioral patterns.
Custom Policy Recommendations
AI processes claims history and client demographics to generate granular risk profiles for insurance products. These models leverage predictive analytics to process driving telemetry, weather data, and vehicle service records, quantifying accident probabilities for auto insurance applicants. This data-driven approach enables highly accurate risk stratification and facilitates the generation of personalized premium quotes.
AI-powered recommendation systems mine customer data and interaction histories to identify optimal insurance product configurations and upselling opportunities tailored to individual risk profiles. The system evaluates parameters such as property dimensions, geolocation data, and installed security features to propose supplementary coverage options, including water damage protection or enhanced theft deterrence. This personalization strategy aims to maximize customer satisfaction metrics while simultaneously driving revenue growth through targeted product offerings.
Generative AI Use Cases for Banking and Finance
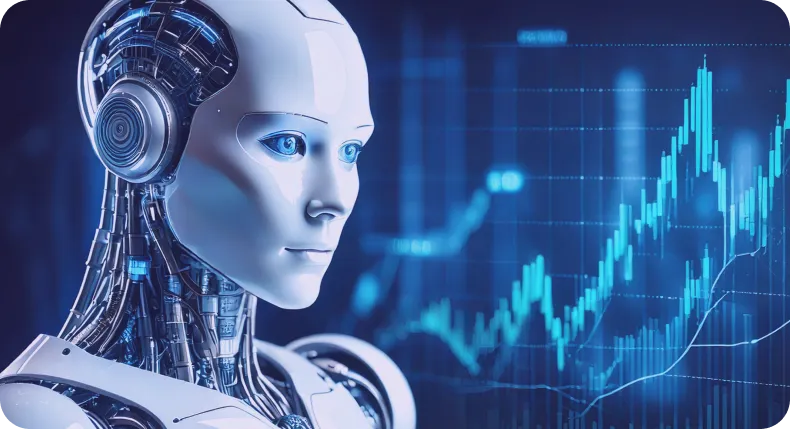
Superior Customer Service
Generative AI-powered conversational agents operate nonstop, offering tailored support for account inquiries, transaction details, and financial services. These systems employ robust authentication protocols to verify user identity before accessing sensitive data. Upon successful authentication, AI swiftly retrieves and presents account balances through secure channels, circumventing the need for traditional online banking interfaces or long customer service queues.
Chatbots leverage natural language understanding to interpret user queries, dynamically accessing relevant databases to fetch requested information. By integrating with core banking systems, these AI agents provide real-time data access, enhancing customer service.
This automated approach not only reduces strain on human support teams but also empowers customers with instant, self-service options for routine banking tasks. AI continually refines its responses based on user interactions, improving its ability to address a wide array of financial inquiries.
Personalized Investment Advice and Management
Generative AI chatbots execute algorithmic financial advice tailored to individual fiscal profiles and risk tolerances. These systems interface with human financial advisors for complex investment planning and wealth management strategies.
When queried about retirement savings, AI analyzes the user's financial metrics and generates investment recommendations based on risk assessment and temporal parameters. The system then facilitates scheduling with a human advisor for personalized consultation, combining automated and expert-driven financial guidance.
Market Analysis and Forecasting
The latest AI algorithms leverage machine learning to process extensive historical financial datasets, market trends, and economic indicators. These systems employ sophisticated statistical models and deep learning techniques to identify complex patterns and correlations.
By analyzing vast quantities of structured and unstructured data, AI models generate predictive insights into market movements and asset performance. Financial institutions utilize these AI-powered forecasts to optimize investment strategies, conduct risk assessments, and enhance portfolio management. The integration of AI in financial analysis enables more precise, data-driven decision-making, leading to improved returns and reduced market volatility exposure.
Custom-Tailored Product Suggestions
AI financial tools employ sophisticated algorithms to analyze multidimensional customer data, historical data, income streams, risk profiles, and market dynamics to identify correlations and patterns within vast datasets. AI then generates highly personalized financial recommendations, tailoring investment portfolios, insurance plans, and credit products to individual client profiles.
By integrating real-time market analysis with customer-specific financial goals and risk tolerances, AI optimizes product suggestions and savings strategies. This tech strategy allows financial institutions to deliver precision-targeted services, increasing customer satisfaction and improving financial outcomes.
Generative AI Use Cases for Design

Collaboration and Brainstorming
Generative AI tools function as collaborative ideation engines, synthesizing design concepts through recursive neural networks trained on extensive visual datasets. These AI agents employ natural language processing to extract key parameters from user inputs, including target demographics, functional requirements, and brand constraints.
The system then activates generative adversarial networks to produce diverse layout permutations, chromatic schemes, and user interface components. It iteratively refines outputs based on real-time designer feedback, leveraging reinforcement learning algorithms to optimize design proposals. This AI-powered approach accelerates the conceptualization phase, presenting designers with an array of viable options that adhere to specified criteria while exploring novel creative territories.
Inspiration and Feedback
Generative AI systems execute multi-modal analysis on uploaded design assets, providing data-driven feedback on visual elements such as chromatic composition, typographic selection, and spatial alignment.
AI applies computer vision algorithms to deconstruct image choices and compare them against vast databases of successful designs. It then synthesizes insights on current design trends, using natural language generation to articulate potential improvements.
The system also employs content-based image retrieval techniques to analyze similar high-performing images and extract salient design features, presenting these as inspirational stimuli to augment the user’s creative process.
Automatic Color Palette Suggestions
Advanced generative AI algorithms synthesize brand identity parameters, demographic psychographics, and competitive landscape analyses to produce ideal color palette suggestions. These systems employ computer vision techniques to analyze existing brand assets and machine learning models to extract color preferences from target audience data. AI integrates this information with color theory principles and current design trends, utilizing generative adversarial networks (GANs) to create novel, brand-aligned chromatic schemes.
Designers leverage these AI-generated palettes to inform visual identity development, ensuring cohesive brand representation across multiple platforms while maintaining market differentiation. This process enhances design efficiency and brand consistency across all applications.
Trend Forecasts
AI-driven trend forecasting systems analyze diverse datasets from social media platforms, fashion events, and consumer behavior studies. These systems utilize deep learning models to identify emerging aesthetic patterns, color schemes, and design motifs across multiple channels. Sophisticated sentiment analysis techniques gauge consumer receptivity to nascent trends.
AI synthesizes these multifaceted inputs to generate predictive models of future design preferences. It then produces actionable insights and trend forecasts, empowering designers to anticipate market shifts and inform strategic design decisions. This data-centric strategy optimizes product development cycles and increases market responsiveness in the fast-paced design industry.
Generative AI Use Cases for Automotive

Dealer Performance Assessment
AI systems perform deep analysis of enterprise data, such as sales metrics, customer sentiment indices, and operational key performance indicators. These AI algorithms ingest structured and unstructured data from sales transactions, survey responses, digital reviews, inventory management systems, and employee performance metrics. AI applies machine learning techniques to identify correlations and patterns, revealing high-performing dealerships based on sales volume and customer satisfaction scores.
AI employs predictive modeling to isolate areas for operational enhancement and generates data-driven recommendations for process optimization. It leverages NLP to extract actionable insights from textual feedback, while time series analysis forecasts future trends. This comprehensive analytical approach enables the AI to formulate targeted growth strategies, prioritizing interventions that promise the highest return on investment across the dealership network.
Service Technician Support
AI actively facilitates real-time diagnostic support for automotive service technicians. These systems analyze vehicular telemetry and maintenance documentation, enabling AI-powered chatbots to generate interactive, sequenced troubleshooting protocols for complex mechanical issues.
This process will reduce operational costs and minimize vehicle downtime in service facilities. The AI algorithms guide technicians through systematic diagnostic procedures, expediting the identification and resolution of mechanical malfunctions with increased precision and rapidity.
Marketing Content Creation
Generative AI systems automate the production of high-impact marketing content across all platforms. These algorithms leverage transformer architectures and reinforcement learning to generate contextually relevant and persuasive copy.
For automotive manufacturers launching electric vehicles, AI content generation systems analyze product specifications, target audience data, and market trends to synthesize compelling headlines, detailed product narratives, and conversion-optimized calls to action. This strategy significantly accelerates content creation workflows, maintains message consistency, and maximizes marketing campaign efficacy through data-driven, audience-tailored communications.
Autonomous Vehicles
Advanced AI systems integrate multi-modal sensor fusion, deep neural networks, and reinforcement learning algorithms to enable autonomous vehicle operation. These systems employ sophisticated computer vision techniques and LiDAR processing to perceive and interpret complex traffic environments in real-time.
Chain-of-thought prompting mechanisms enhance decision-making processes, allowing the AI to generate and evaluate multiple potential actions based on current traffic conditions, vehicular dynamics, and predicted behaviors of other road users. This technology facilitates nuanced navigation through intricate scenarios, optimizing route planning and collision avoidance. The AI continuously refines its decision-making models through iterative learning, progressively increasing safety and operational efficiency in all driving conditions.
Generative AI Uses for Healthcare
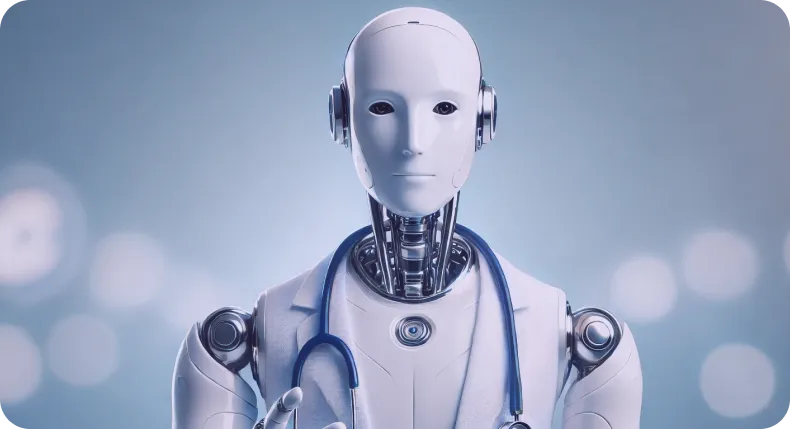
Symptom Monitoring
Healthcare AI executes preliminary symptom triage, addresses fundamental health inquiries, and routes patients to suitable medical resources. When a patient presents with acute thoracic discomfort and engages a Gen AI chatbot, the system initiates a comprehensive symptom evaluation protocol.
It administers a targeted questionnaire to ascertain pain intensity and associated clinical manifestations. AI rapidly analyzes the patient's responses, employing decision-tree algorithms to determine the appropriate course of action.
Based on this analysis, the system either issues an urgent recommendation for immediate medical intervention in cases of potentially life-threatening conditions, or prescribes self-care measures for milder symptomatic presentations. This automated triage process optimizes resource allocation and expedites critical care delivery when warranted.
Appointment Booking and Notifications
Artificial intelligence uses advanced scheduling algorithms to optimize appointment management, dispatch automated reminders, and validate attendance, mitigating administrative overhead for healthcare institutions. These systems leverage natural language processing and a machine learning model to interpret and act upon patient requests in real-time.
When a patient initiates a rescheduling request, the AI chatbot instantaneously accesses the provider's calendar system, applies constraint satisfaction algorithms to identify suitable time slots, and executes the rescheduling operation. This process eliminates synchronous communication via telephone, reduces wait times, and improves operational efficiency by managing complex scheduling logic across multiple specialists or departments.
AI Image Analysis
Advanced convolutional neural networks and deep learning algorithms process medical imaging data, including radiographs, computed tomography, and magnetic resonance images, to detect and classify anatomical anomalies. These AI systems employ image segmentation techniques and feature extraction methods to isolate regions of interest and identify potential pathologies.
ML models trained on vast datasets of annotated medical images enable rapid anomaly detection and quantification. AI generates detailed heatmaps and probability scores for suspicious lesions or tumors, facilitating prioritized review by healthcare professionals. This computer-aided detection and diagnosis system augments clinical decision-making processes, potentially improving diagnostic accuracy and expediting patient triage.
Custom Search Results
Machine learning algorithms, employing collaborative filtering and content-based recommendation systems, dynamically personalize search results for medical professionals. These platforms leverage natural language processing to analyze user query patterns, research focus areas, and patient demographic data. The system integrates this information with real-time updates from clinical trial databases and medical literature repositories.
Using reinforcement learning techniques, AI continuously refines its relevance ranking algorithms, optimizing information retrieval for individual users. This approach implements a sophisticated vector space model to match user profiles with multidimensional content features to suggest highly targeted and timely search results. This technology promotes information discovery efficiency and provides clinical decision support for healthcare practitioners.
Generative AI Use Cases for Legal
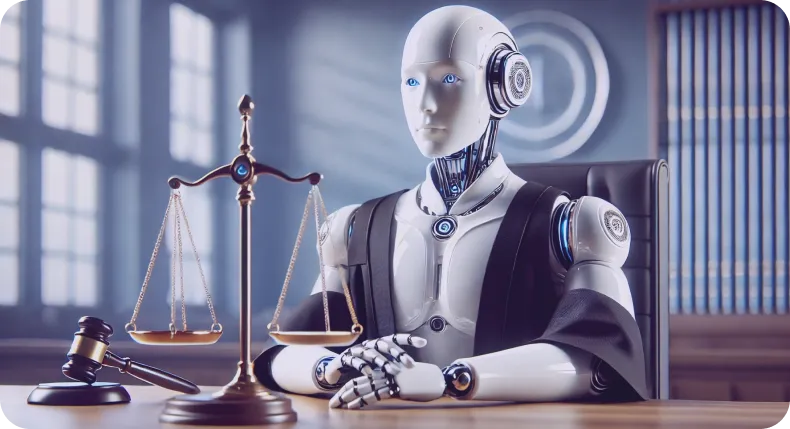
24-Hour Assistance
AI chatbots leverage legal information retrieval algorithms to disseminate juridical data, address high-frequency inquiries, and route users to pertinent resources at any time of the day or night. These systems parse queries and match them against a comprehensive legal knowledge base.
The chatbot instantiates topic-specific dialogue trees for common legal issues, generating responses and pulling up relevant legal forms and self-help documentation. It implements conditional logic to facilitate handoffs to human representatives during operational hours, maintaining continuity of service and escalation of complex inquiries.
Automated Document Review
Legal AI chatbots utilize advanced document analysis algorithms to scrutinize legal instruments, flagging potential anomalies, discrepancies, and sections necessitating human expert examination. These systems understand complex legal language and identify critical elements within documents.
AI performs semantic analysis on contracts, leases, and various legal texts, isolating clauses that demand heightened scrutiny. It applies risk assessment models to highlight potential legal vulnerabilities and employs pattern recognition to detect inconsistencies across document sections. The system then generates a prioritized list of flagged items for subsequent review by legal professionals, optimizing the document evaluation process.
Efficient Legal Research
Advanced natural language processing models parse complex legal queries, employing semantic analysis to discern search intent. These AI systems leverage deep learning algorithms to process extensive legal corpora, including case law, statutes, and judicial opinions. Utilizing vector space models and transformer architectures, AI identifies and ranks relevant legal precedents based on multifaceted similarity metrics.
The system implements named-entity recognition and relationship extraction techniques to pull up pertinent case details and legal principles. For patent infringement cases, AI employs specialized ontologies and machine learning classifiers to identify analogous technological disputes and relevant intellectual property rulings. AI-assisted legal research significantly accelerates the process and increases precision and comprehensiveness during case preparation.
Automated Legal Billing
Gen AI streamlines legal billing by automating time tracking, invoice generation, and error detection. It actively analyzes task durations, such as drafting briefs or attending hearings, to produce accurate invoices, minimizing manual input and reducing the likelihood of billing errors. AI enhances efficiency and lowers the administrative workload for legal teams.
Generative AI Use Cases for Retail
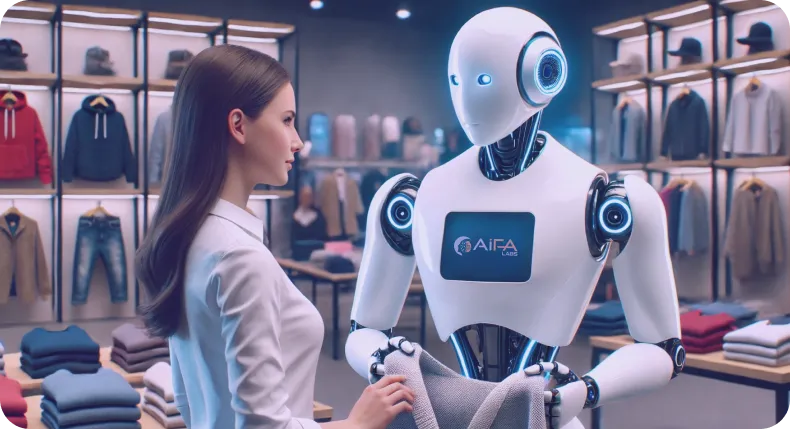
Search Trend Analysis
Generative AI systems execute advanced analytics algorithms on search trend data and customer interaction patterns to isolate high-demand products, detect nascent search queries, and diagnose potential search functionality anomalies. This analysis empowers e-commerce platforms to refine their search engines and curate product portfolios more effectively.
The AI implements natural language processing and trend forecasting models to extract actionable insights from search data. Retailers leverage these insights to dynamically optimize product metadata, refine taxonomies, and restructure category hierarchies. This data-driven approach enhances product discoverability, aligning inventory presentation with evolving consumer search behaviors and market demands.
Semantic Search
AI systems employ advanced natural language understanding (NLU) algorithms to decode semantic intent and contextual nuances underlying customer queries, transcending simplistic keyword matching. These systems utilize deep learning models and semantic networks to establish conceptual relationships between search terms and product attributes.
When processing a query such as "dress shirt for work," retail AI executes intent classification and entity recognition tasks. It then maps the derived intent to a broader product taxonomy, potentially surfacing results from categories like "formal tops" or "professional button-ups." This semantic search capability enables the system to return highly relevant product recommendations that align with the user's implicit needs, even when explicit keyword matches are absent.
Product Recognition
Gen AI leverages image and video recognition to power visual search and personalized product recommendations. By analyzing uploaded images or videos, the AI system identifies similar products and suggests complementary items based on visual features and related data, enhancing the accuracy and relevance of recommendations.
Custom Product Recommendations
Gen AI analyzes customer purchase history, browsing patterns, and demographic data to deliver personalized product recommendations. By matching customer behavior with relevant products, the AI system enhances upselling and cross-selling opportunities, leading to higher customer satisfaction and increased sales.
Generative AI Use Cases for Human Resources
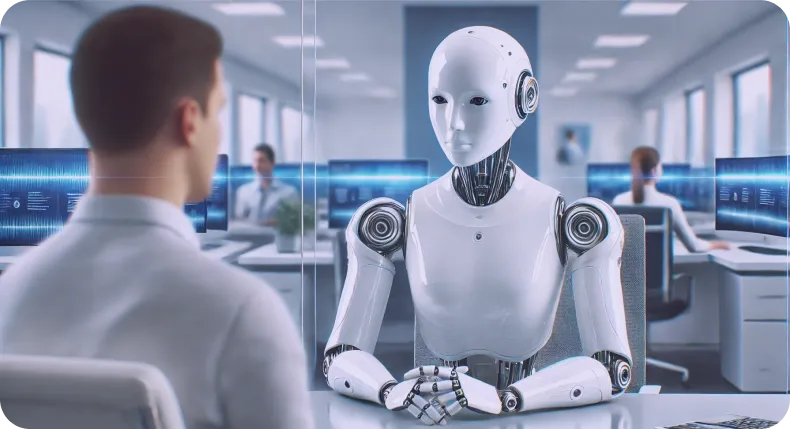
Automated Scheduling
AI models optimize workforce schedules by leveraging operational data analytics and constraint-based modeling. These systems automate intraday management processes, reducing administrative overhead and maintaining optimal staffing levels, even with fluctuating production volumes or shifting customer traffic patterns. This process automation increases operational efficiency and minimizes labor expenditures.
Performance Evaluations
HR departments can leverage AI-powered tools to monitor and quantify employee performance metrics based on multimodal data streams. AI performance management platforms aggregate and analyze task completion rates, project contribution levels, and peer evaluation data.
These systems generate comprehensive performance analytics reports for managerial review, delineating core competencies, development areas, and longitudinal productivity trends. This data-driven approach facilitates objective, equitable performance assessments, mitigating potential biases in the evaluation process
Workforce Optimization
AI tools can analyze multidimensional workforce datasets to forecast future talent acquisition requirements. The algorithms synthesize current skill inventories, industry trend projections, and organizational growth trajectories to generate predictive hiring models.
By leveraging machine learning techniques, the system extrapolates role-specific demand curves for upcoming fiscal periods. This enables C-suite HR executives to implement proactive talent acquisition strategies, ensuring optimal workforce composition for current and future strategic business objectives.
Enhanced Recruiting
Generative AI systems revolutionize talent acquisition processes by employing natural language processing algorithms to parse and analyze candidate résumés against predefined job requisitions. These AI-driven applicant tracking systems (ATS) utilize semantic matching and ML models to quantify candidate-job fit scores.
The platforms generate ranked shortlists of optimal candidates, significantly reducing time-to-hire metrics and minimizing human bias in the initial screening phase. By automating the résumé evaluation process, these systems ensure comprehensive and consistent candidate assessments, thereby optimizing the efficiency and efficacy of the recruitment funnel.
Generative AI Use Cases for eCommerce
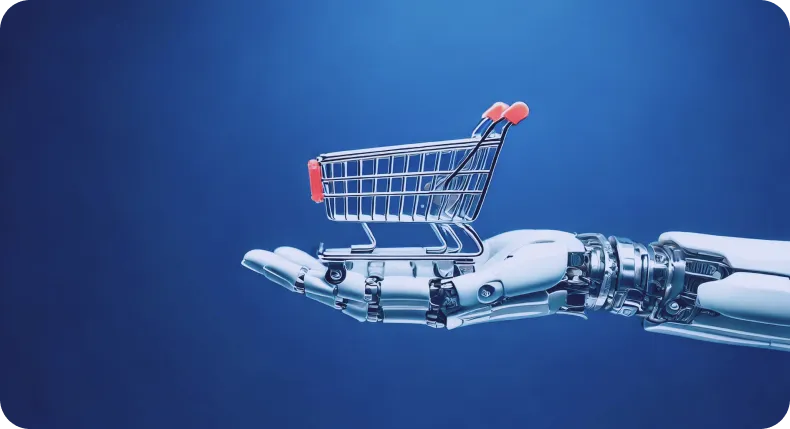
Demand Forecasting and Inventory Management
Many companies are utilizing AI to perform multivariate time series analysis with predictive modeling algorithms to forecast product-specific demand curves. These models process several data streams, including historical sales metrics, market trend indicators, and exogenous variables, to optimize inventory levels and mitigate stock-out or overstock scenarios.
An AI-powered demand forecasting engine can integrate meteorological predictions, social media sentiment analysis, and longitudinal sales data to anticipate demand surges for seasonal products. For instance, the system may predict elevated sunglasses demand preceding the summer season. This predictive capability enables retailers to implement just-in-time inventory management strategies, preemptively adjusting stock levels to meet projected demand spikes.
By leveraging machine learning algorithms for continuous demand sensing and forecasting, the system minimizes lost sales opportunities due to stock-outs while concurrently reducing excess inventory carrying costs. This data-driven approach to inventory management optimizes working capital allocation and enhances overall supply chain efficiency.
Real-Time Pricing
Some AI tools use dynamic pricing algorithms that integrate real-time market data streams, competitive intelligence, and customer behavior analytics to optimize pricing strategies and generate targeted promotional offers. These AI-powered pricing engines maximize profitability metrics while increasing customer satisfaction.
In the context of online travel services, AI-powered revenue management systems deploy machine learning models to execute real-time price adjustments for accommodation offerings. These models ingest and process multidimensional data sets, including:
- Real-time demand fluctuations
- Competitor rate structures
- Individual customer preference profiles
- Historical booking patterns
The system leverages predictive analytics to dynamically calibrate pricing, maintaining optimal competitive positioning while maximizing yield. Simultaneously, the AI engine employs collaborative filtering and recommender systems to generate personalized deal structures, aligning offers with individual customer budget constraints and travel requirements.
This AI-powered approach to price optimization and offer personalization enables travel platforms to implement a granular, customer-centric pricing strategy. The system continuously iterates and refines its models, adapting to evolving market conditions and customer behaviors, maintaining a sustained competitive advantage and customer value proposition.
Make Artificial Intelligence Work for Your Business | AiFA Labs
Need help with the integration of generative AI into your business? The Cerebro Generative AI Platform makes it easier than ever. As an all-in-one AI solution tailored for each individual client, Cerebro increases productivity and efficiency in every industry. Book a free demonstration online or call us at (469) 864-6370