Discover how artificial intelligence (AI) and the Internet of Things (IoT) have joined forces to change the world in which we live. The artificial intelligence of things promises to reshape our personal lives and almost every industry in the economy. But what is AIoT, and what does it do? Let’s explore the benefits and use cases of AI and IoT.
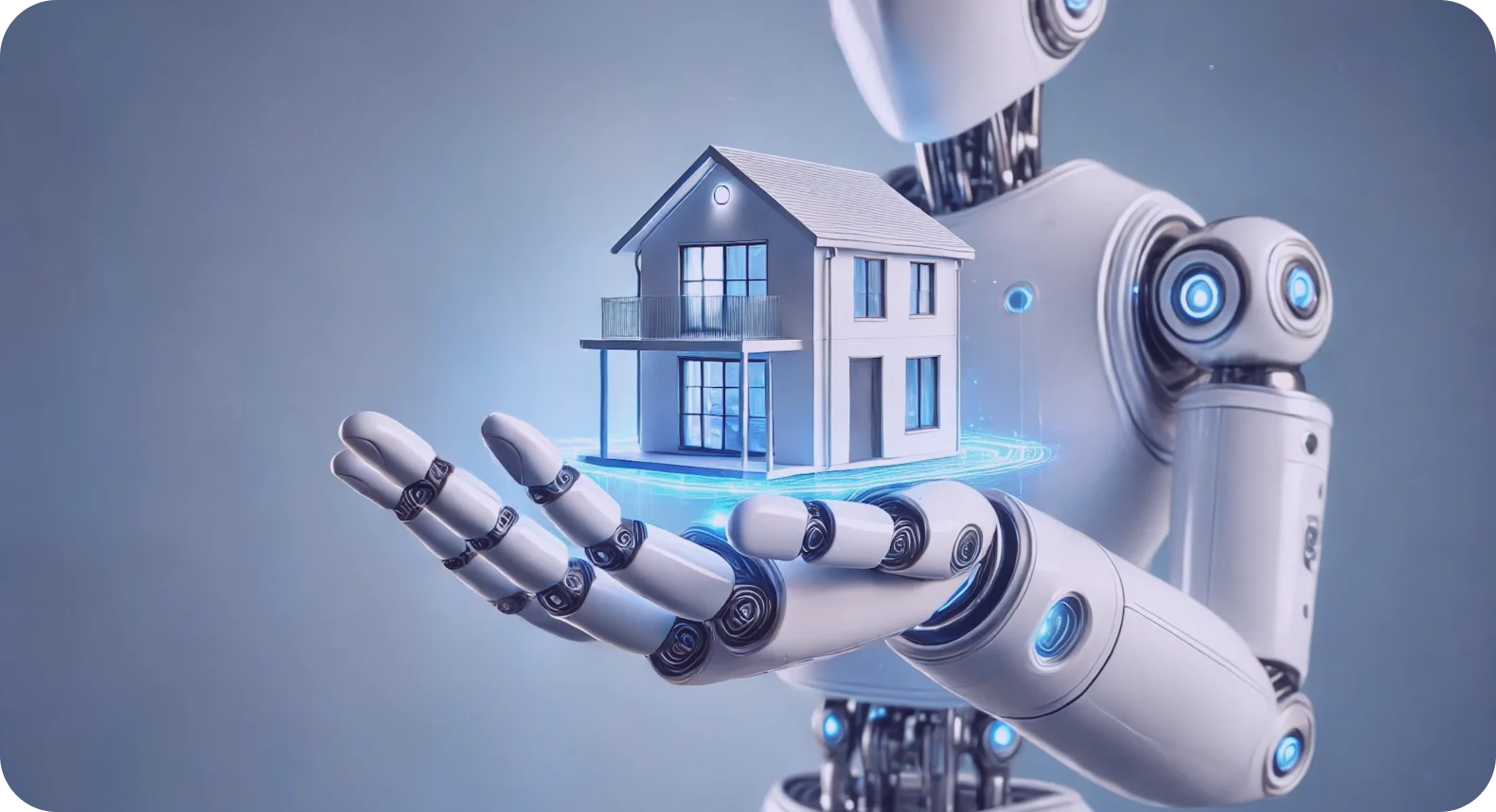
What Is Artificial Intelligence of Things (AIoT)?
Artificial intelligence of things (AIoT) is the amalgamation of artificial intelligence (AI) and the Internet of Things (IoT) to support advanced data management, seamless human-AI interactions, and cutting-edge IoT devices. AIoT combines the latest AI systems with state-of-the-art IoT infrastructure.
Artificial Intelligence
Artificial intelligence, or AI, simulates human intelligence through computer vision, speech recognition, machine learning, natural language processing, and other technologies. Some of the most popular smart systems improve operational efficiency by automatically performing otherwise laborious manual tasks such as data collection, processing, and analysis. AI can also detect anomalies in human behavior, perform quality control, and drive autonomous vehicles.
Internet of Things
The Internet of Things is a system of connected IoT devices, mechanical machines, digital appliances, and other goods that can transfer data over the internet without human intervention. IoT devices create a significant amount of data by using software and sensors to monitor and track the performance of anything from automobiles to coffee machines.
The Objective of Artificial Intelligence Internet of Things
The goal of combining AI with IoT is to facilitate more efficient IoT functions, ease human-machine interactions, optimize data management, and enhance data analysis. AI leverages its machine learning capabilities to maximize the effectiveness of the IoT. By integrating AI with IoT, many of the devices connected to the internet can analyze data to predict and mitigate potential issues, adjust system operations on the fly, and self-improve through deep learning.

What Does AIoT Do?
AIoT devices integrate AI algorithms directly into infrastructure components, including firmware and specialized chipsets, interconnected via IoT protocols. APIs facilitate seamless interoperability among hardware, software, and platform elements, enabling transparent communication and operation.
Once deployed, IoT sensors generate and aggregate data, which AI algorithms subsequently process to extract actionable insights and optimize operational efficiency. AI systems leverage machine learning (ML) techniques to derive these insights from the collected data.
AIoT architectures predominantly appear in the cloud or at the edge.
Edge Computing and AIoT
Edge computing has emerged as a pivotal technology in recent years, catalyzing the advancement of IoT, 5G, and AI technologies. As the primary driver of AIoT, edge computing shifts data processing from centralized cloud infrastructures to network peripheries.

Edge-Computing-Powered Artificial Intelligence of Things
Edge computing architectures facilitate distributed system designs that prioritize on-device data processing, delivering high efficiency, scalability, robustness, and low-latency performance. At first, machine learning and deep learning (DL) operations were confined to cloud environments due to their computational intensity and resource demands.
Now, edge-computing AIoT enables efficient execution of computationally demanding AIoT applications at network edges, satisfying the stringent processing speed and latency requirements of the top IoT use cases.
On-Device Machine Learning in AIoT Ecosystems
The latest advancements in ML algorithms and hardware have accelerated the proliferation of interconnected, intelligent, and adaptive devices across critical infrastructure sectors, including healthcare, agriculture, transportation, and logistics.
The migration of AI processing from cloud-centric models to distributed edge devices mitigates bottlenecks, reduces latency, and addresses privacy concerns inherent in cloud-based AI implementations.
AIoT deployments require modern edge devices with sufficient computational resources for ML tasks that traditional low-power IoT endpoints can not perform. Still, edge devices inherently face constraints in resource capacity and power budgets. Consequently, AIoT apps must factor in the hardware cost, AI model optimization, performance, and application design efficiency.
This challenge has driven recent trends toward AI model compression and efficacy enhancement. Techniques such as model quantization, pruning, and knowledge distillation enable low-latency, energy-efficient inference at the edge. The resulting ML models can execute on resource-constrained devices like embedded computers, smartphones, and systems-on-chips (SoCs).
Embedded machine learning transforms AIoT devices into autonomous, intelligent systems capable of independent data processing. The convergence of several advancements across a wide range of industries has facilitated the efficient application of AI technologies in edge environments. The resultant flexibility and scalability of AIoT systems enable the development of previously infeasible real-world applications, pushing the boundaries of intelligent edge computing.
Cloud Computing and AIoT
Cloud-based IoT leverages cloud computing platforms to manage and process data streams from IoT endpoints. Integrating IoT devices with cloud infrastructure is crucial because it facilitates data storage, processing, and accessibility for many applications and services. Cloud-based AIoT architectures comprise four distinct layers.

1. User Interface Layer
The user interface layer comprises web portals and mobile applications that serve as the primary interaction points for end-users, enabling data visualization, system control, and configuration management.
2. Device Layer
The device layer encompasses an array of hardware components, including RFID tags, Bluetooth Low Energy (BLE) beacons, multi-modal sensors, connected vehicles, industrial automation equipment, embedded systems, and biometric monitoring devices.
3. Connectivity Layer
The connectivity layer incorporates field and cloud gateways, consisting of hardware or software elements that establish bidirectional communication channels between cloud storage repositories and edge devices such as sensors, controllers, and other intelligent endpoints.
4. Cloud Layer
The cloud layer executes data processing via AI engines, implements scalable data storage solutions, generates data visualizations, performs advanced analytics, and provides data access through RESTful or GraphQL APIs.
10 Benefits of Combining AI and IOT
Artificial Intelligence in IoT offers several benefits to companies that wish to increase efficiency, reduce costs, and manage risks. Let’s examine some advantages that companies enjoy by leveraging the artificial intelligence of things.
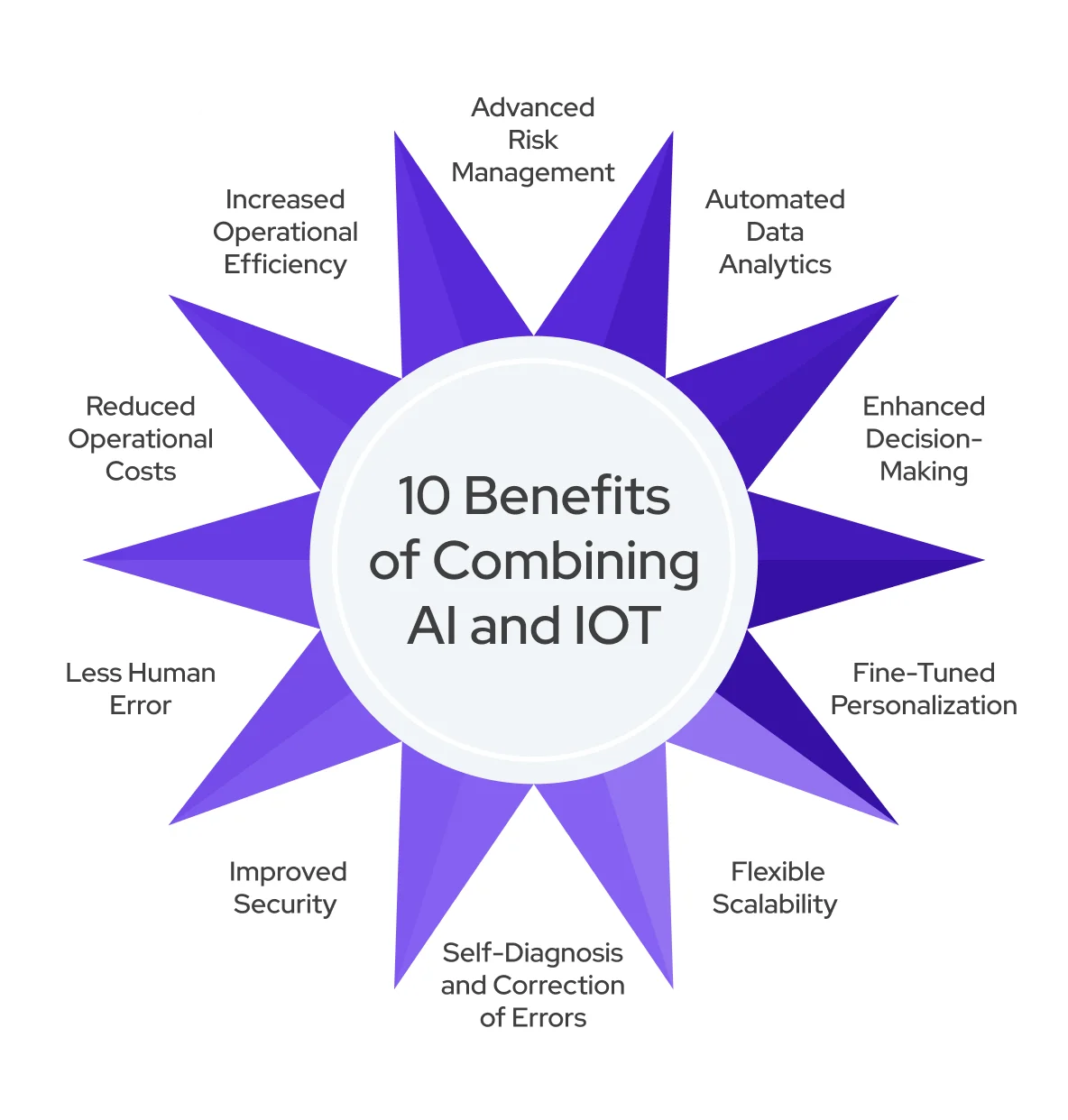
1. Advanced Risk Management
Distributed AIoT architectures perform predictive risk mitigation through real-time analysis of critical parameters and workplace safety metrics. Organizations leverage these systems for proactive threat management, while insurers deploy them for dynamic risk assessment of industrial equipment and manufacturing facilities.
2. Automated Data Analytics
AIoT systems autonomously execute complex data analytics tasks, liberating human resources from routine device monitoring. Machine learning algorithms continuously process sensor data streams, automate pattern recognition, and generate actionable insights, optimizing operational efficiency and reducing labor costs.
3. Enhanced Decision-Making
AI algorithms execute high-throughput processing of IoT sensor streams, extracting actionable insights that drive informed decision-making. In clinical applications, AI systems analyze biometric data from wearable devices to detect early disease indicators and predict adverse health events. Agricultural implementations leverage AI to process sensor arrays for crop yield optimization through precision farming techniques.
4. Fine-Tuned Personalization
AIoT systems synthesize behavioral telemetry data to generate personalized user experiences through adaptive machine learning algorithms. Smart audio systems leverage AI to construct user preference models from interaction patterns, supporting automated curation of context-aware content recommendations.

5. Flexible Scalability
AIoT architectures enable dynamic scalability by seamlessly integrating additional sensor nodes and edge devices, facilitating process optimization and feature expansion. This modular approach supports progressive system enhancement while maintaining network efficiency and data processing capabilities.
6. Improved Security
Machine learning algorithms actively monitor IoT endpoints for security vulnerabilities, employing anomaly detection to identify potential threats through real-time sensor data analysis. For example, computer vision systems process surveillance feeds to detect behavioral anomalies and trigger automated security response protocols.
7. Increased Operational Efficiency
AIoT systems employ adaptive machine learning algorithms to extract temporal patterns and performance metrics from sensor data streams, enabling autonomous optimization of system parameters. This continuous feedback loop facilitates dynamic resource allocation and operational refinements to maximize efficiency across connected infrastructure.
8. Less Human Error
AIoT architectures mitigate financial losses from human error by processing data at source through edge computing and machine learning algorithms. This integrated approach eliminates traditional multi-phase workflows and intermediary touchpoints, significantly reducing data entry errors and transmission vulnerabilities.
9. Reduced Operational Costs
AIoT systems optimize resource utilization through intelligent automation, exemplified by occupancy-based environmental control systems in smart buildings. In industrial settings, sensor arrays and computer vision systems enable predictive maintenance protocols, preventing equipment failures and minimizing operational disruptions through real-time condition monitoring.
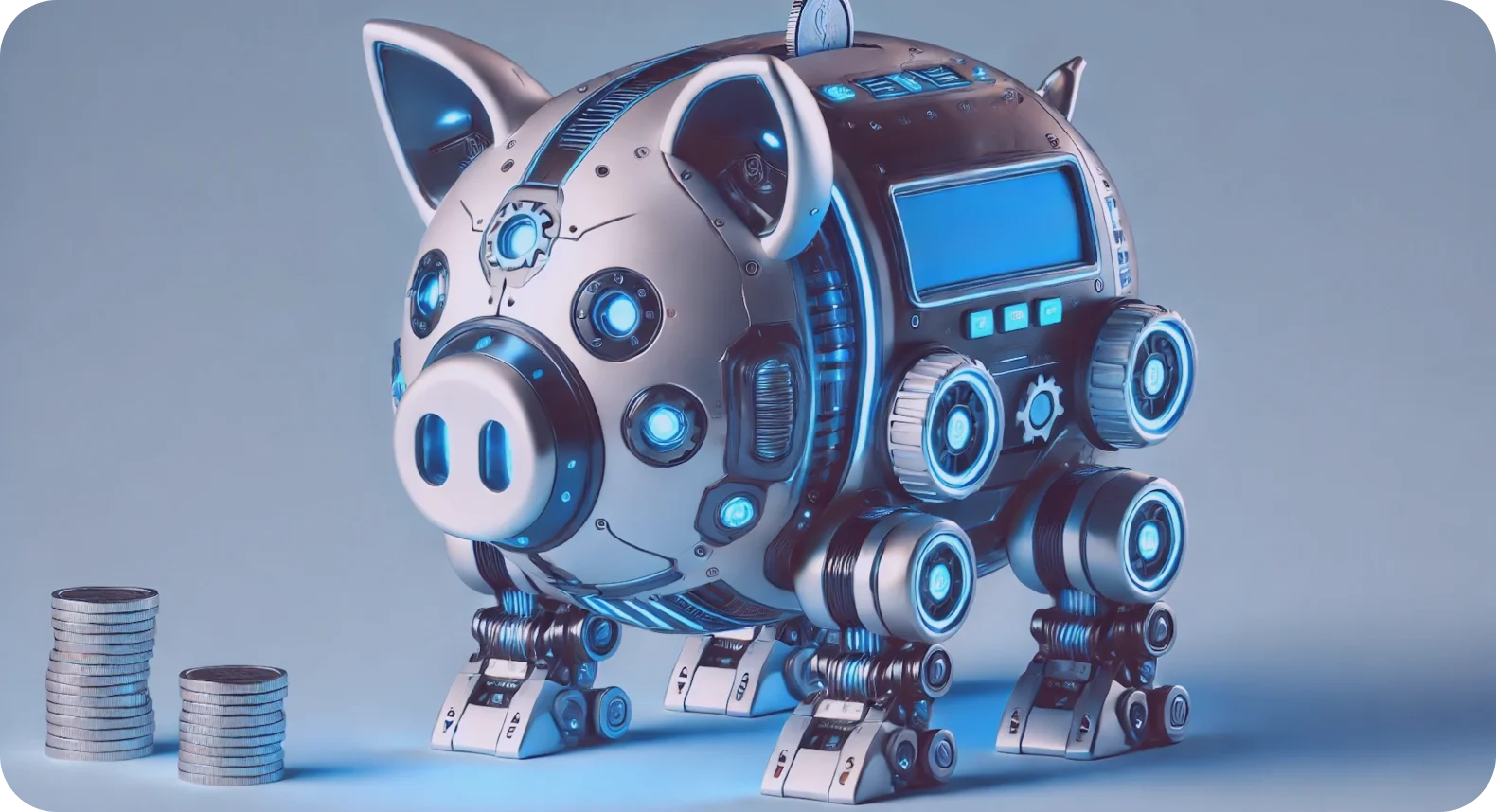
10. Self-Diagnosis and Correction of Errors
AIoT systems leverage real-time analytics to detect performance anomalies and system vulnerabilities, enabling dynamic self-optimization through automated feedback loops. This adaptive architecture allows for autonomous adjustments to operational parameters, ensuring continuous system reliability and efficiency.
Use Cases for AIoT
The Internet of Things and artificial intelligence have come together to unlock new use cases for these advanced technologies. From agriculture to smart factories, the list of use cases for AIoT continues to expand. Some of the top AIoT applications include:
- Agriculture — Optimizes resource use, predicts potential issues, and enables smarter, real-time agricultural decisions
- Automated Vehicles — Uses sensors and cameras to drive safely without human intervention, leveraging real-time data analysis and edge AI to make accurate driving decisions without offloading data to the cloud
- Healthcare — Performs remote patient monitoring, personalized care, and predictive analysis of health data to identify potential issues early
- Human Resources — Integrates with social media and HR platforms to analyze any data collected and assist professionals in decision-making processes
- Logistics — Collects environmental data, such as from a warehouse, and utilizes AI to make navigation decisions for robots
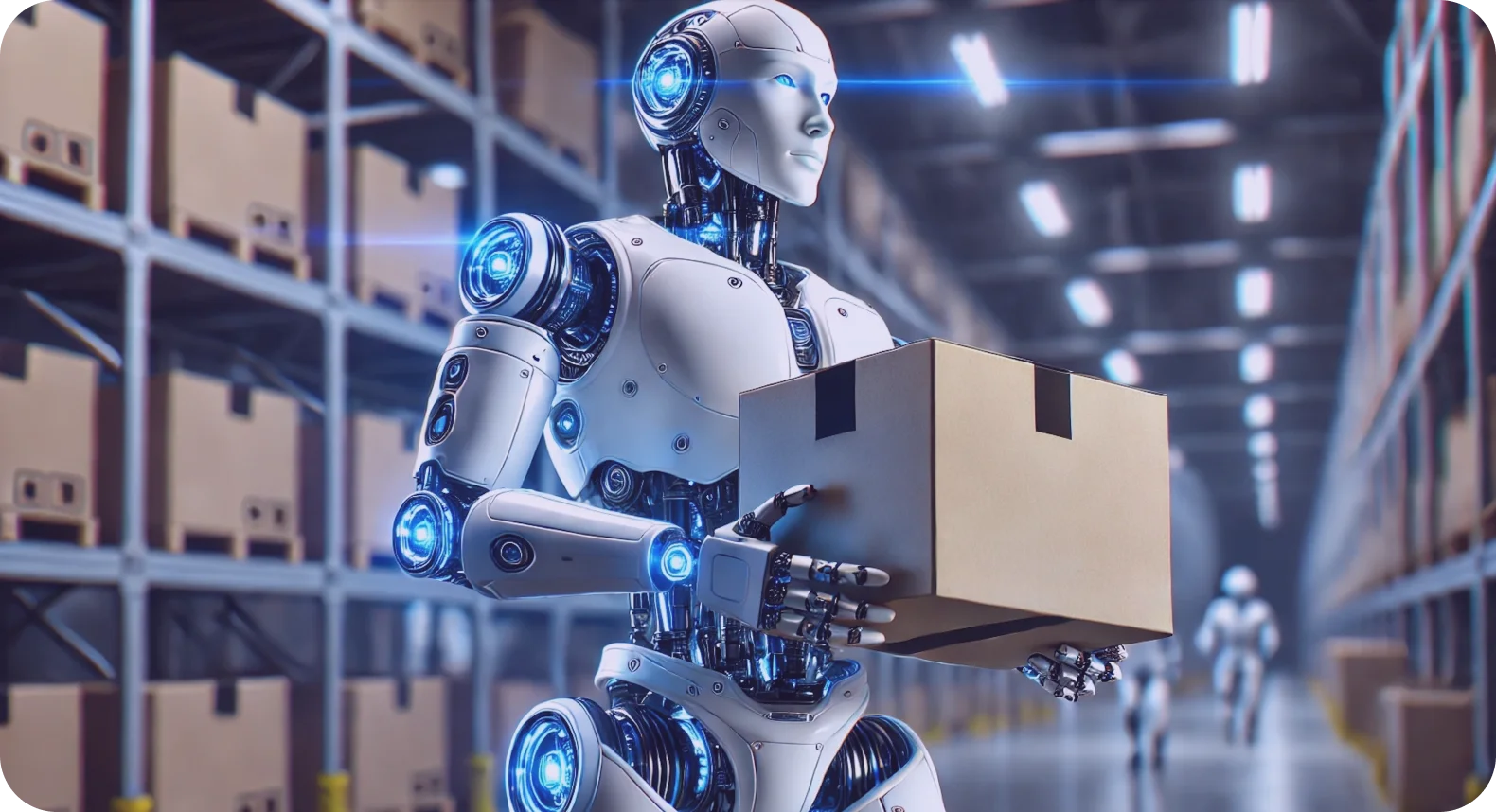
- Predictive Maintenance — Analyzes IoT sensor data and predicts machine failures, allowing businesses to prevent downtime and reduce maintenance costs through proactive measures
- Robotics — Assists in manufacturing and assembly by leveraging IoT data and AI tools, such as computer vision, for tasks like production, packaging, and quality control
- Smart Cities — Leverages sensors, lights, and meters to collect data that enhances operational efficiency, drives economic growth, and improves residents’ quality of life
- Smart Factories — Executes real-time machinery monitoring and defect detection by replacing traditional machine vision with deep learning models that analyze video feeds to identify defective parts
- Smart Homes — Learn from human interaction and user data to provide personalized, customized support for smart appliances based on user habits
- Smart Office Buildings — Uses environmental sensors to optimize lighting and temperature for energy savings, and facial recognition technology for automated access control
- Smart Retail — Identifies shoppers with smart cameras and verifies whether they scanned their items at self-checkout before exiting the store
- Traffic Monitoring — Performs real-time traffic analysis using smart drones for congestion and crowd analysis, while computer vision systems detect accidents, traffic violations, and stopped vehicles
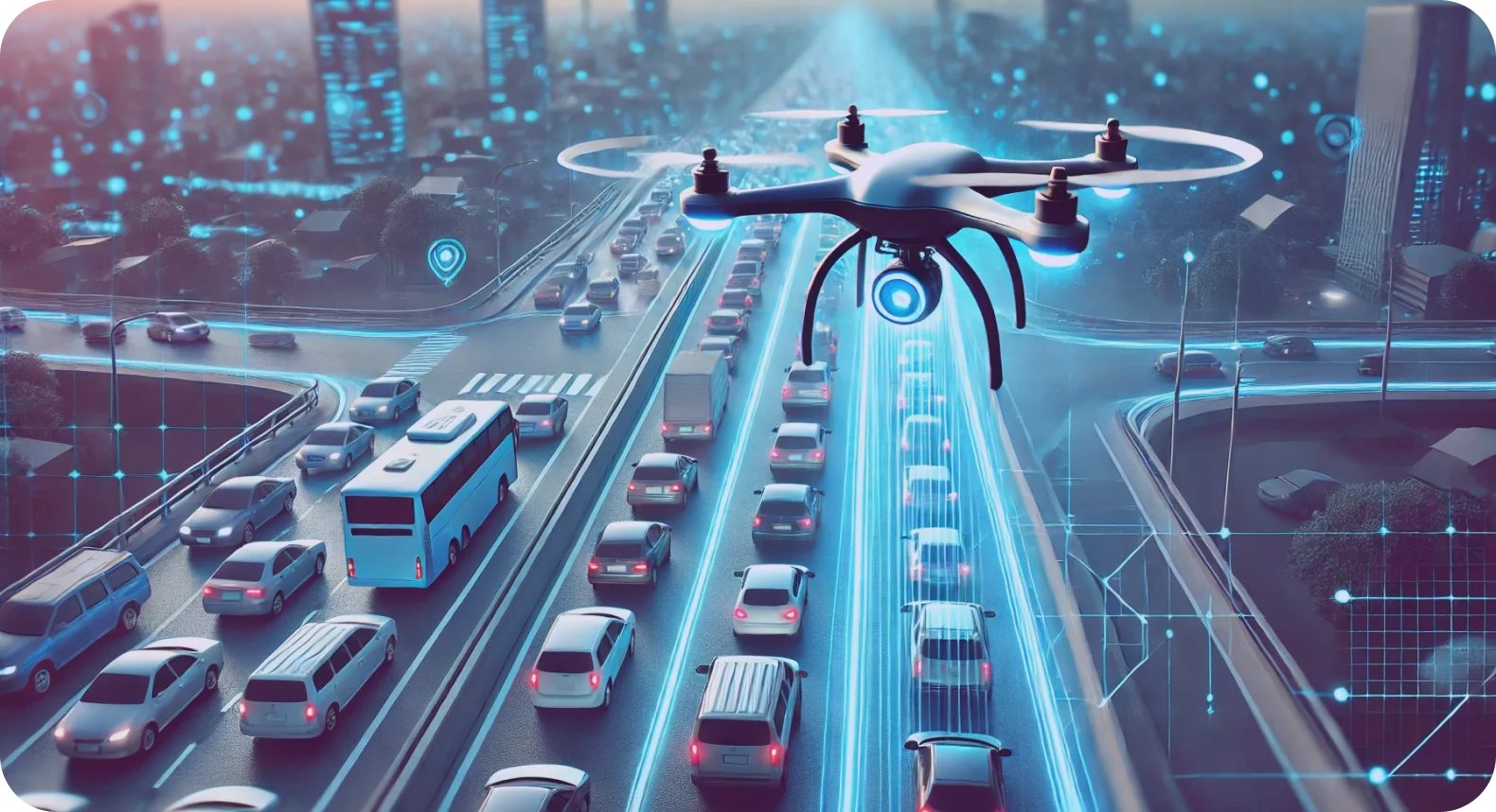
- Video Surveillance — Enhances video surveillance and security by analyzing real-time camera feeds, automatically detecting objects and recognizing events
- Wearable Devices — Monitors and evaluates personal health data to provide insights into an individual’s fitness, sleep patterns, and overall well-being
Be a Part of IOT and AI Future Developments | AiFA Labs
Now that you know what AIoT means, see what the AI of things can do for your business. With the Cerebro Generative AI Platform, artificial intelligence, IoT, and automation work together to prevent mistakes and help things run more smoothly. Book a free demonstration of Cerebro today or call AiFA Labs at (469) 864-6370.