Artificial intelligence (AI) and machine learning (ML) are poised to transform problem-solving and decision-making across diverse fields, including healthcare, finance, social media, and self-driving vehicles. Yet, like all technologies, AI and ML are susceptible to bias, which can have profound implications for society. Let’s explore what AI bias is and examine a few different examples.
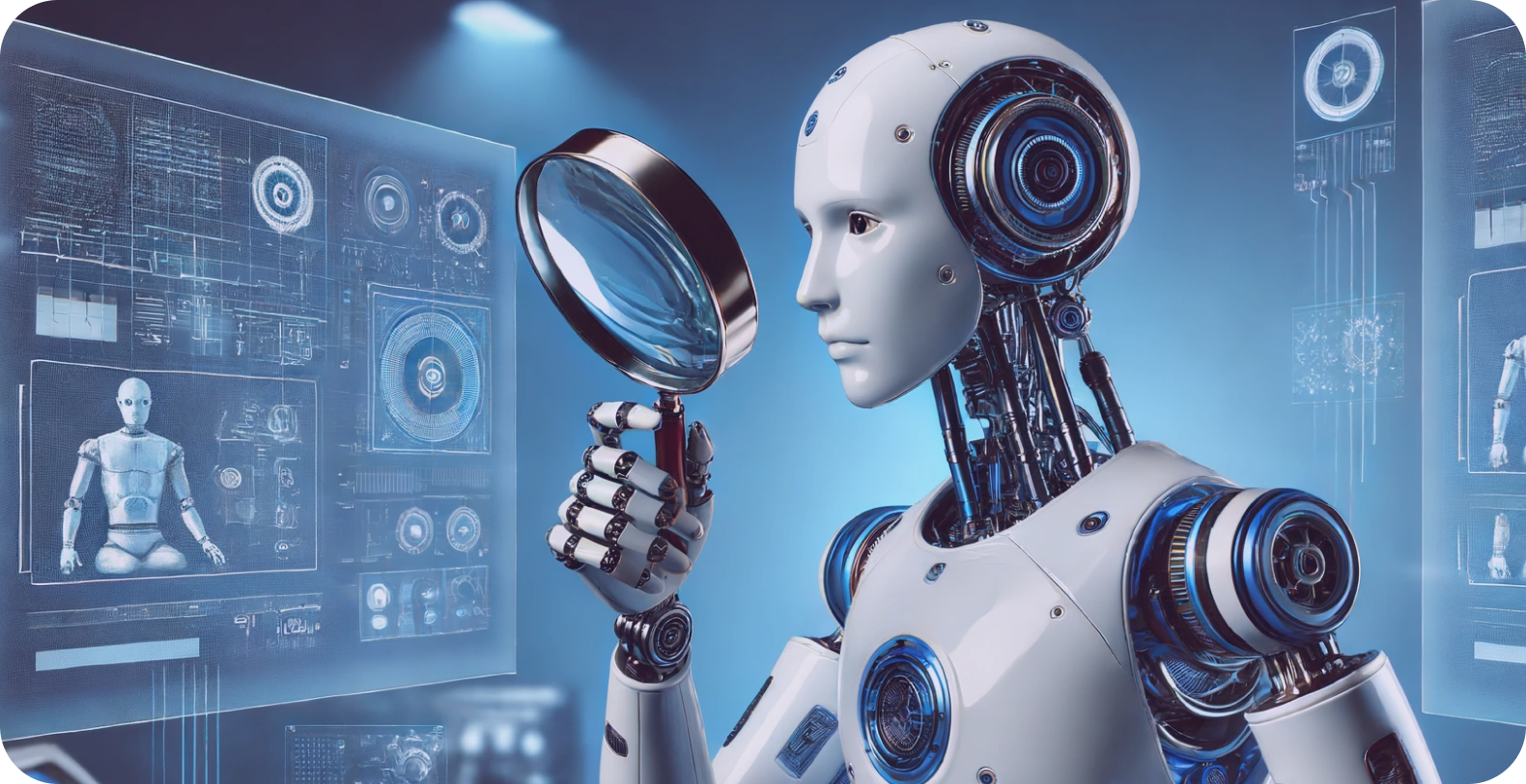
What Is AI Bias?
AI bias is when an AI model regularly produces inaccurate output due to flawed training. When a biased model trains on a dataset, it generates significant mistakes. Bias can infiltrate a machine learning project at various steps in the process.
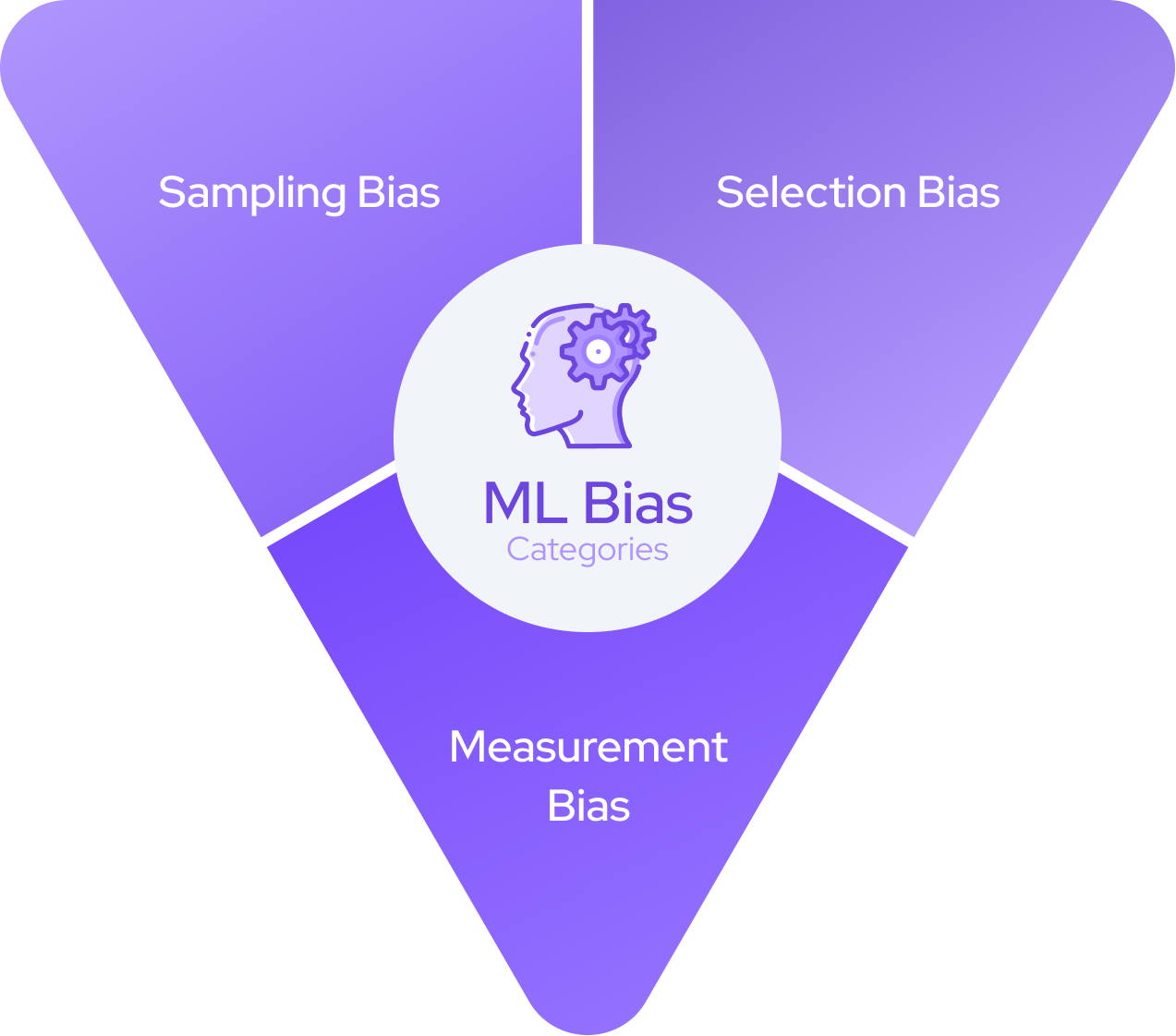
Key Categories of ML Bias
- Sampling Bias: This type of bias occurs when training data inaccurately represents the target population or the issue at hand. It can arise from non-random sample selection or when sub-groups are disproportionately represented.
- Selection Bias: This kind of bias emerges when the training data is skewed due to irregular data collection or sampling methods. It may occur when samples are chosen based on human biases or when data is not randomly collected.
- Measurement Bias: This category of bias relates to data errors from flaws in the data collection or measurement process. It can stem from faulty measurement tools, human error, or inconsistencies in measurement.
Understanding and addressing these biases is crucial for developing AI systems that are fair, equitable, and beneficial to all segments of society.
Significance of Bias in Machine Learning
ML bias can have profound impacts on society, especially when it influences critical decisions like hiring, firing, lending, or administering medicine. Discover the importance of reducing bias in machine learning.
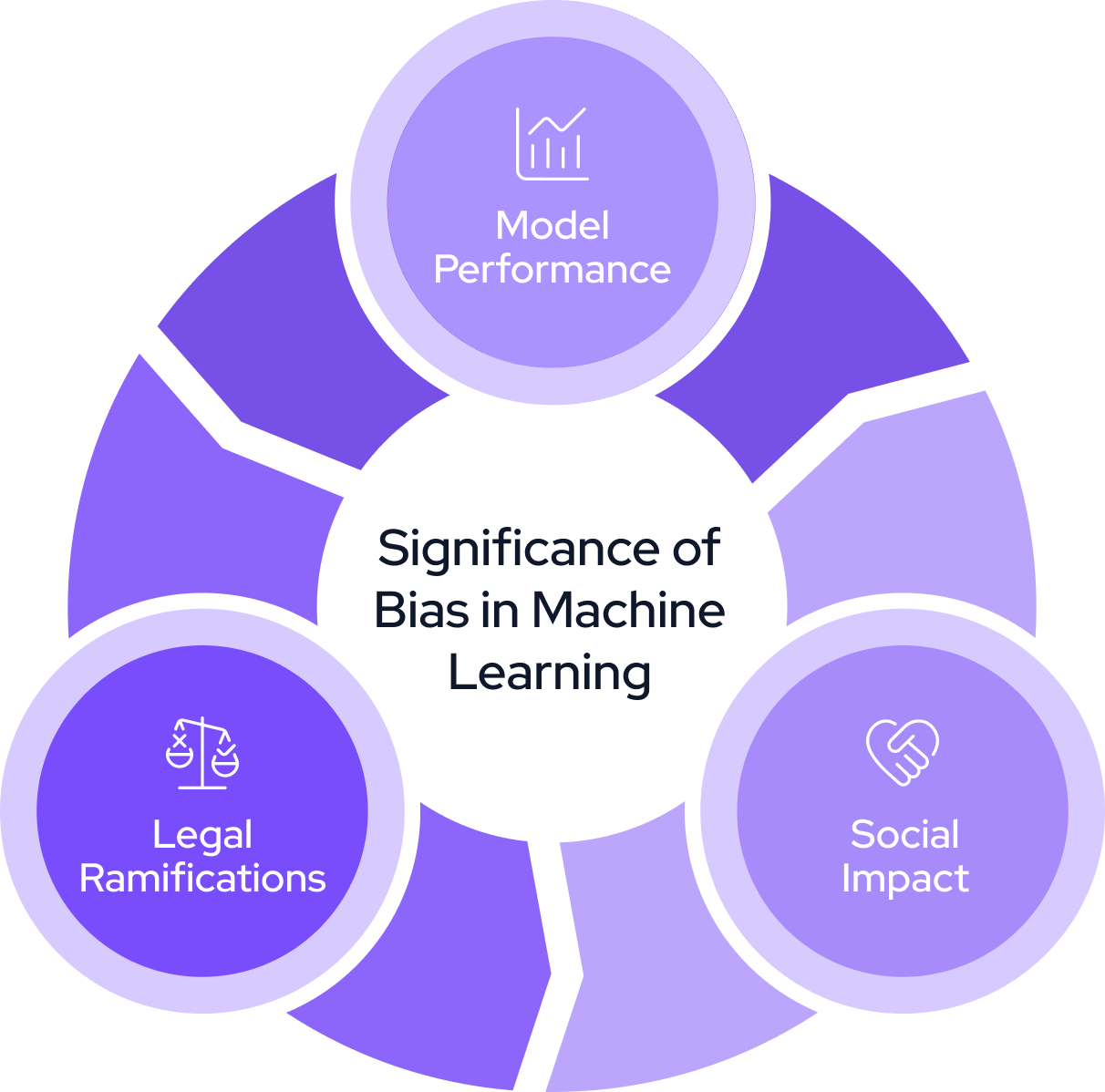
Model Performance
Bias amplifies errors in analytics, making the models less accurate and effective. Inaccurate predictions or distorted outputs can lead to significant repercussions, particularly in sensitive fields like medicine and law.
Legal Ramifications
Companies may face legal issues due to unlawful treatment of protected groups based on their heritage, language, appearance, and other characteristics. These discriminatory practices can also damage a company's reputation.
Social Impact
When datasets are disproportionately weighted, it can dramatically alter the course of a person’s career, finances, and family life. Biased AI models may promote inequitable results in mortgage approvals, employment applications, and credit card applications.
Given these implications, it's crucial to recognize ML bias and implement systems for detection and mitigation.
Regulatory Developments Concerning AI Bias
Legislative and administrative actions concerning AI bias are becoming more frequent, with policymakers set on allowing only ethical, precise, and unbiased AI models.

Laws Concerning AI Bias in the United States
In the US, AI in recruitment and employment is the most regulated AI use case. For instance, New York, Illinois, Maryland, and other states have enacted regulations on automated employment decision tools (AEDTs) that use AI for applicant screening and other employment matters.
New York's Local Law 144 of 2021 mandates employers to conduct bias audits on AEDTs, make audit results public, and notify employees or job candidates about the use of AEDTs. The Equal Opportunity Employment Commission (EEOC) also has a program overseeing "algorithmic fairness" in hiring.
Laws Concerning AI Bias in the EU
The EU Agency for Fundamental Rights (FRA) published a report in December 2022 calling for algorithm assessments, including testing for bias, delivering guidelines for private data, identifying racial and gender biases, promoting language diversity, and enhancing fact-based administration of the technology.
Preventing Bias in Generative AI | Cerebro by AiFA Labs
Addressing bias in AI calls for teamwork among lawmakers, software developers, and scientific researchers to recommend laws pertaining to AI bias. At AiFA Labs, we have made significant progress in eliminating bias from the Cerebro Generative AI Platform. Book a free demo online or call us at (469) 864-6370 for more information.