Natural language processing (NLP) stands is a remarkable branch of artificial intelligence that enables computers to grasp, analyze, and produce human language. NLP has significant applications in various fields, including healthcare, human resources, retail, and e-commerce.
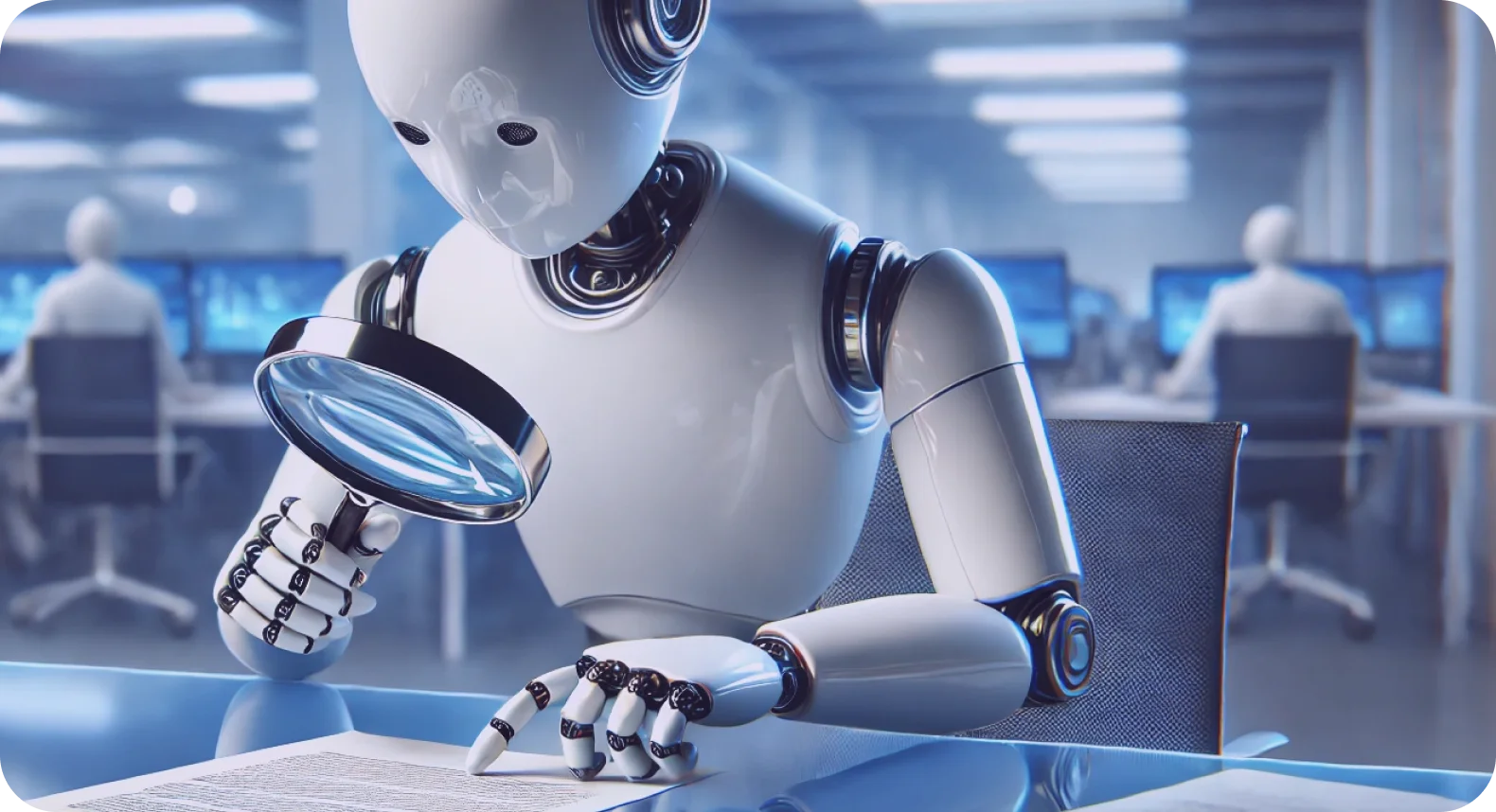
NLP supports how we communicate with digital systems, fostering more seamless, natural interactions. It lies at the forefront of a technological shift, fundamentally changing our engagement with computers. Below, find eight distinct examples illustrating how NLP tasks enrich our everyday experiences and drive progress in several industries.
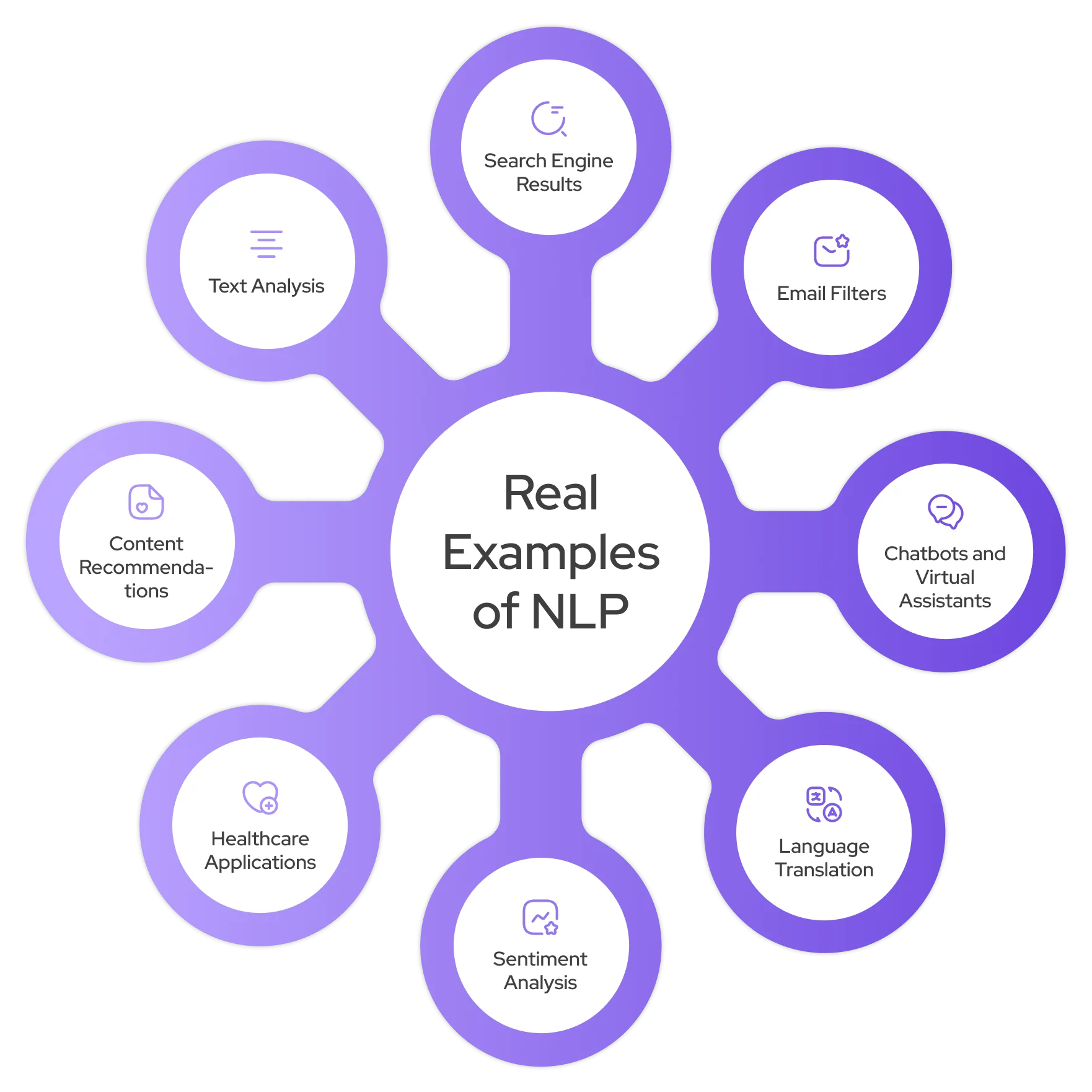
1. Email Filters
Common natural language processing examples, such as spam detection, harness AI technologies and computational linguistics to optimize business operations. Through keyword extraction and word sense disambiguation (WSD), these systems sift through vast amounts of text data to pinpoint relevant messages while filtering out unwanted content.
By effectively identifying and blocking spam emails, these natural language processing tools significantly enrich the user experience. It results in a clutter-free inbox, empowering users to focus on important communications without the distraction of irrelevant messages. NLP transforms the way we interact with digital information, making our online experiences more streamlined and productive.

Advanced Spam Detection Techniques
Modern email services like Gmail tap into advanced natural language processing technologies to effectively combat spam and phishing attempts. By analyzing the context in which words are used, Gmail can uncover sophisticated spam tactics that traditional filters might miss, successfully identifying deceptive emails.
Intelligent Sorting for Improved User Experience
To enhance user experience, NLP techniques automatically categorize incoming messages into groups, such as primary, social, and promotions. When users mark emails as spam, the system learns from these actions, refining its algorithms to improve filtering accuracy over time. Gmail employs proactive security measures, such as alerts for suspicious emails, empowering users to navigate their inboxes with confidence and avoid potential phishing threats.
2. Search Engine Results
Natural language processing (NLP) is essential for supporting search engine capabilities, enabling users to access relevant results. Modern search engines like Google employ NLP to analyze and interpret user queries. One NLP search example is when a user types “restaurants near me” into a search engine, and the system interprets the request to provide a list of local dining options. This advanced functionality simplifies the search experience and intuitively addresses user needs.

Understanding User Intent and Context
A major advantage of NLP in search engines lies in its capacity to comprehend the context of a query. Instead of focusing exclusively on the precise words entered, the system interprets certain words on a broader spectrum. This flexibility allows users to express their queries in their own words.
Some natural language search engine examples include Google’s search algorithms, which understand queries in everyday language. By grasping user intent and contextual nuances, NLP-powered search engines deliver more accurate information, streamlining the navigation of search engine results pages (SERPs). This capability augments the search experience, making it more intuitive and user-friendly, enabling users to easily locate the information they require.
Predictive Text and Speech Recognition
Predictive text and speech recognition improve user interactions by anticipating inputs and accurately interpreting spoken language. They connect ambiguous queries to relevant information, ensuring users receive precise, meaningful results tailored to their specific needs.
3. Language Translation
To gain a comprehensive understanding of human language, translation systems utilize a natural language toolkit. This toolkit includes a specialized programming language that enhances language processing and helps translate text from one language to another. By integrating advanced algorithms with a vast data volume, it becomes possible to improve the accuracy of translations.
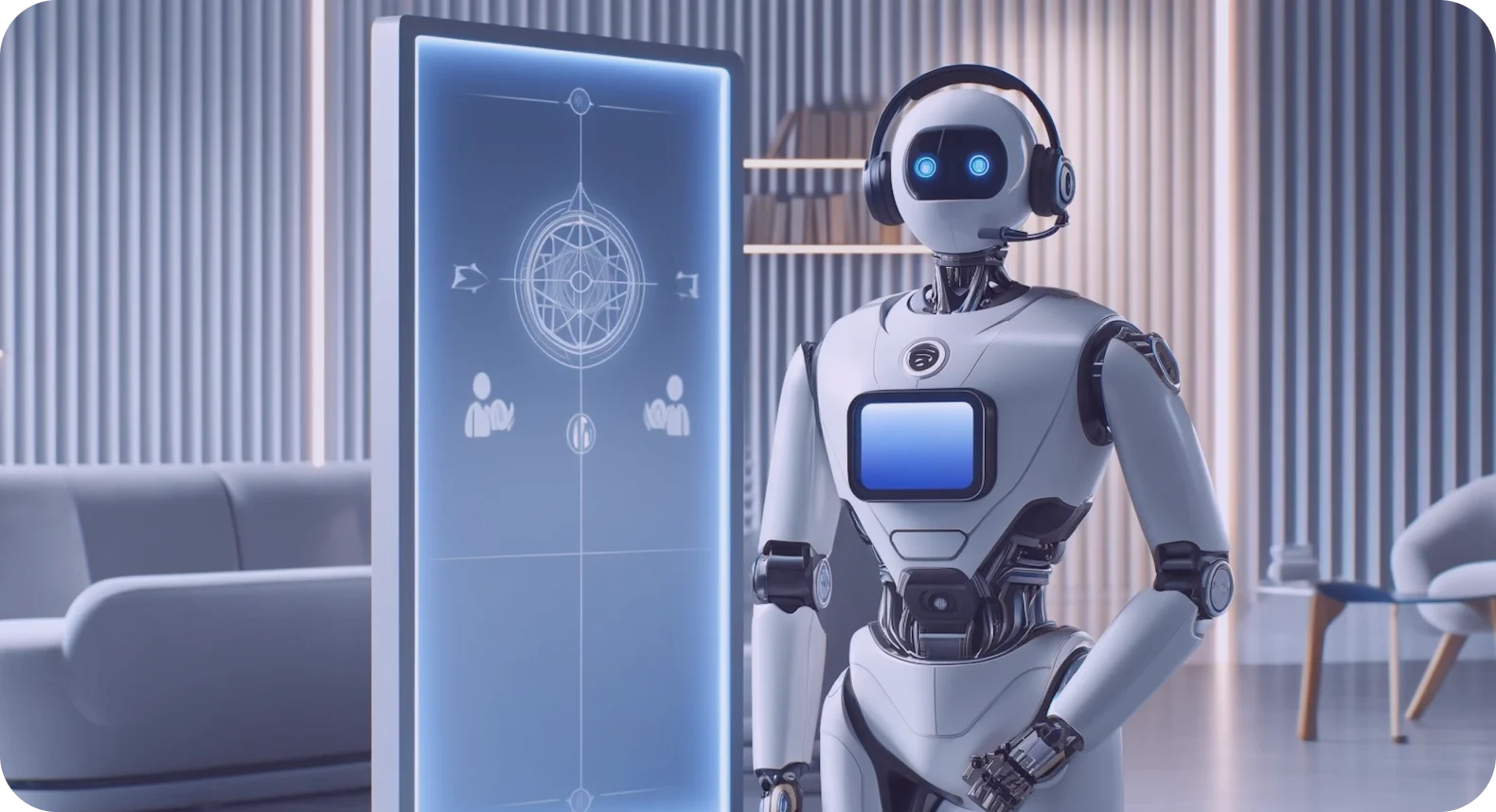
Machine Translation Systems
Machine translation systems, like Google Translate, leverage advanced NLP technologies to deliver instantaneous text translations modified to user requirements. By employing state-of-the-art deep learning models, these systems grasp the contextual meaning of sentences, facilitating smooth communication in multiple languages without human intervention.
A key advancement in translation accuracy involves the improved handling of grammatical rules. In the past, direct translations frequently yielded clumsy, grammatically flawed results. However, today’s advanced NLP tools excel at producing translations that adhere to grammatical standards and capture the rich subtleties of the target language, bridging gaps between languages with remarkable clarity.
Streamlining the Translation Process
Modern translation tools possess the ability to automatically understand human language in the input text, facilitating seamless transitions between languages. This innovation eliminates the need for users to manually specify the source language, streamlining the translation process. As a result, users enjoy a simplified experience, allowing them to focus on communication rather than navigating technical details.
4. Sentiment Analysis
Natural language processing examples include sentiment analysis tools that analyze customer feedback and automated translation services that convert text from one language to another while preserving the meaning.
AI and natural language understanding technologies optimize the capabilities of NLP systems, allowing them to better understand context, meaning, and relationships within text. This deeper understanding leads to more nuanced interpretation and analysis, improving the effectiveness of language processing.
Central to this evolution is text analytics, which translates unstructured text data into actionable insights through a blend of linguistic, statistical, and machine-learning methodologies. For instance, sentiment analysis employs these NLP techniques to evaluate social media interactions and customer feedback, equipping businesses with a clearer understanding of their audience.
By utilizing machine learning algorithms, organizations can interpret user intent and evaluate customer satisfaction, providing valuable insights that contribute to the improvement of products and services. These developments reimagine how businesses connect with their audiences, fostering more informed, responsive engagement strategies.

Categorizing Sentiments for Strategic Insights
Sentiment analysis remains one of the most essential NLP examples for businesses seeking actionable insights. It typically categorizes sentiments into positive, negative, or neutral. This categorization helps organizations quickly spot emerging issues, assess customer satisfaction, and adapt their strategies, as needed. A company can promptly investigate and address concerns, demonstrating its dedication to customer satisfaction.
As a critical application of NLP, sentiment analysis helps businesses connect with customer emotions and preferences. This use case is instrumental in refining decision-making processes and improving the overall customer journey.
Enhancing Customer Relationships
Sentiment analysis helps strengthen customer relationships by allowing businesses to interact with their audience more effectively. By examining sentiments in customer reviews and feedback, companies can tailor their communication approaches to increase engagement. This proactive strategy cultivates customer loyalty and encourages continuous improvement in their offerings.
5. Chatbots and Virtual Assistants
Unlike traditional programming languages that require precision and strict syntax, NLP allows users to communicate in everyday language, making technology more accessible to everyone. AI voice assistants like Siri and Alexa use NLP to understand and respond to human speech, fundamentally transforming our interactions with technology.
Through advanced voice recognition and natural language understanding, these intelligent assistants streamline a wide range of daily tasks, from setting reminders to answering inquiries. Their capability to grasp context allows them to deliver relevant, timely responses, creating a more seamless, engaging user experience. This evolution in human-AI interactions is very convenient and redefines how we engage with digital technology in our everyday lives.
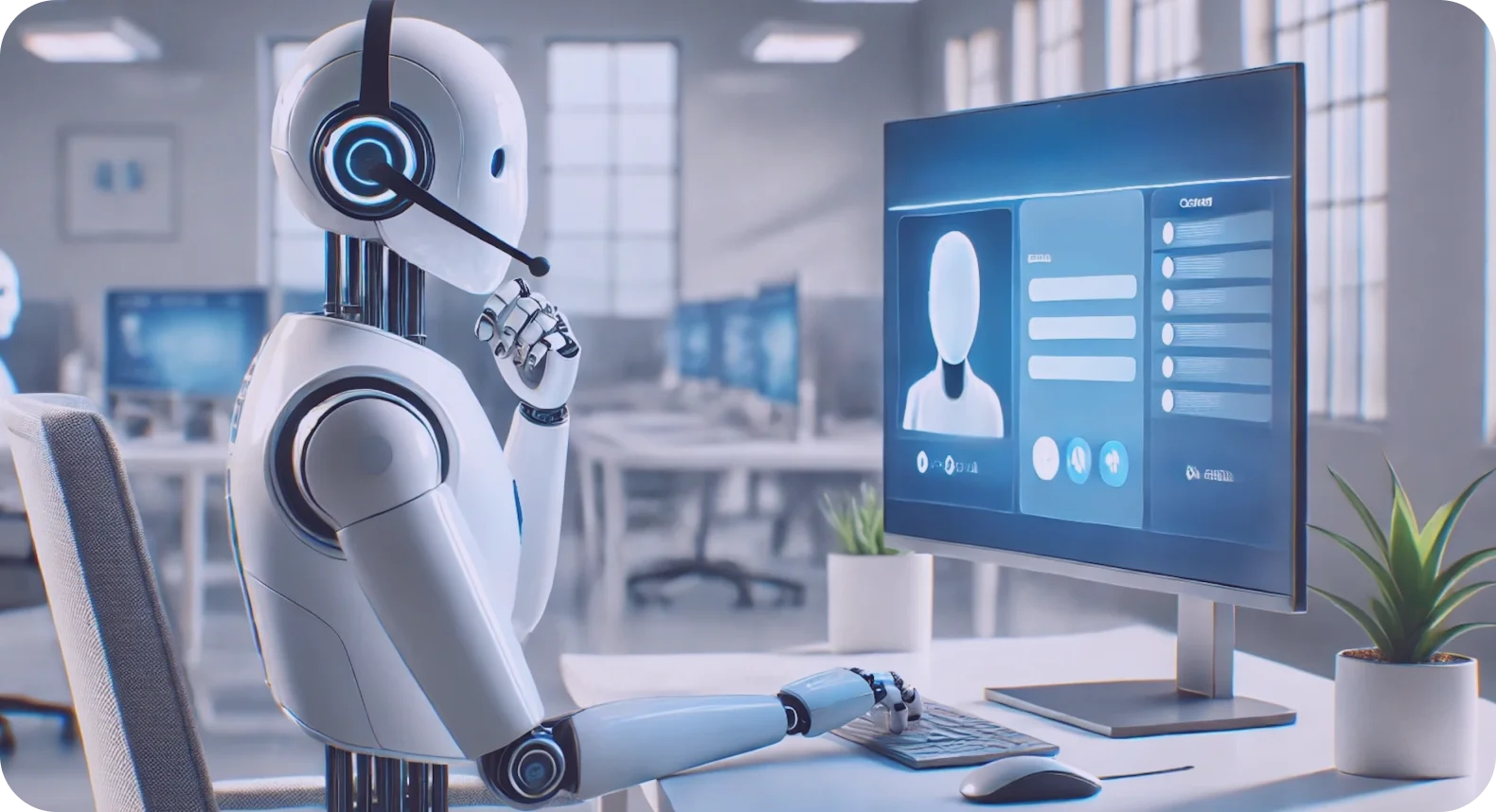
Efficient Customer Engagement
Businesses continue to integrate chatbots powered by large language models to increase the efficiency of customer inquiry management. These advanced chatbots analyze human language to provide prompt, relevant responses, improving the customer experience while streamlining operations.
By learning from previous interactions, these chatbots continually refine their capabilities and understand language by adapting users’ unique needs and preferences. This evolution boosts their effectiveness and curates more personalized, engaging interactions for customers, making chatbots an invaluable asset in modern customer service strategies.
Seamless Integration in Daily Life
Smart assistants like Apple’s Siri and Amazon’s Alexa utilize speech pattern recognition to infer meaning and respond appropriately to user commands. When users initiate interactions with phrases like “Hey Siri” or “Alexa,” they expect the AI voice assistant to understand their requests and provide relevant information based on contextual cues.
As these applications become increasingly integrated into our thermostats, light switches, and vehicles, our interactions with them become more natural. By gathering and analyzing data on individual preferences and behaviors, these assistants create opportunities for deeper, more meaningful engagements.
6. Content Recommendations
Content recommendations based on natural language processing analyze user behavior to suggest relevant articles, products, or media tailored to individual interests.

User Profiling
A fundamental component of the content recommendation process is user profiling, where NLP analyzes interactions such as clicks, likes, and comments to build a comprehensive understanding of user preferences. These business insights empower systems to deliver personalized recommendations that resonate with users.
NLP employs techniques like cosine similarity and word embeddings to evaluate how closely different pieces of content are related. This capability allows systems to identify and recommend items that align with a user’s interests.
Text analysis plays a vital role in topic modeling, keyword extraction, and sentiment analysis. These techniques enable systems to grasp the underlying themes and emotions within the content, resulting in more accurate, relevant suggestions. Collaborative filtering improves the recommendation process by factoring in the preferences of similar users and refining suggestions based on textual feedback and reviews.
Personalization and Dynamic Learning
NLP customizes suggestions based on the nuances and context of user queries, ensuring recommendations feel both engaging and relevant. Additionally, the principle of dynamic learning enables NLP algorithms to continuously adapt by recalling past user interactions.
7. Healthcare Applications
Natural language processing (NLP) has several applications in healthcare, significantly improving patient care, optimizing operational efficiency, and advancing research initiatives. By utilizing NLP, healthcare providers can improve communication, streamline administrative processes, and extract valuable insights from extensive unstructured data. This strategy ultimately fosters more informed decision-making and improves patient outcomes.

Clinical Documentation
One of the primary applications of NLP in healthcare is clinical documentation. NLP automates the documentation process by transcribing and summarizing physician notes, significantly reducing the administrative burden on healthcare providers. This automation ensures patient records are accurate and comprehensive, allowing physicians to devote more time to patient care than paperwork.
In clinical decision support, NLP tools analyze medical literature, clinical guidelines, and patient data to assist healthcare professionals in making informed decisions. By extracting pertinent information from extensive sources, these tools enhance diagnostics and treatment planning, ultimately contributing to better results for patients.
Patient Interaction
Another significant application of NLP involves patient interactions. Chatbots and virtual assistants powered by NLP can effectively engage with patients for various purposes, including scheduling appointments, conducting symptom assessments, and addressing common inquiries. This technology enhances patient access to information and improves overall satisfaction by delivering timely responses and support, ultimately fostering a more seamless healthcare experience.
8. Text Analysis
NLP executes several essential tasks, such as word segmentation, which is crucial for analyzing and comprehending text data. NLP tools excel at processing large volumes of text from various sources, including social media posts, customer reviews, and internal documents, helping organizations extract meaningful insights.
By using techniques like named entity recognition and semantic search, organizations can uncover valuable business insights that inform strategic decision-making and boost overall performance. These NLP capabilities empower companies to identify trends, monitor public sentiment, and gain a deeper understanding of customer needs, ultimately driving more informed actions and improved outputs.

Text Summarization
One of the most significant applications of NLP is text summarization, a technique that condenses lengthy documents and articles into concise summaries, encouraging rapid comprehension of essential information. By employing advanced algorithms that analyze context and extract key themes, NLP systems can generate summaries that highlight critical points while preserving the original message’s intent.
Text summarization offers substantial advantages in today’s fast-paced environment, where information overload is increasingly prevalent. Organizations can leverage NLP-powered summarization to distill reports, research papers, and news articles, allowing employees to stay informed without dedicating excessive time to reading. This feature increases productivity and promotes quicker, more informed decision-making, leading to a more agile, responsive organization.
Enhancing User Experience
Beyond optimizing business processes, NLP text summarization augments the user experience in a range of applications, including content curation and news aggregation. By delivering concise summaries, these tools enable users to absorb information more effectively, allowing them to concentrate on the most relevant content.
Enjoy a Free Demo Example of NLP at Work | AiFA Labs
NLP technology is found in various tools for tasks like language translation, social media monitoring, and processing unstructured data. These systems support a better understanding of human language and facilitate more relatable natural language generation.
The applications of NLP are transformative, bettering our interactions with technology and one another. NLP drives innovation in many industries by automating tasks and providing more profound insights. As organizations recognize its value, the potential for NLP continues to grow, enhancing communication and user experiences. Experience the power of natural language processing firsthand by booking a free demo online or calling AiFA Labs at (469) 864-6370.