Explore how artificial intelligence can learn on its own. As the public starts to learn what AI is and how to use it, people will also discover the various forms of AI. Self-learning AI trains itself on new data in either supervised or unsupervised settings. It also learns from positive and negative reinforcement from users. Let’s examine the different types of self-learning artificial intelligence and its benefits.
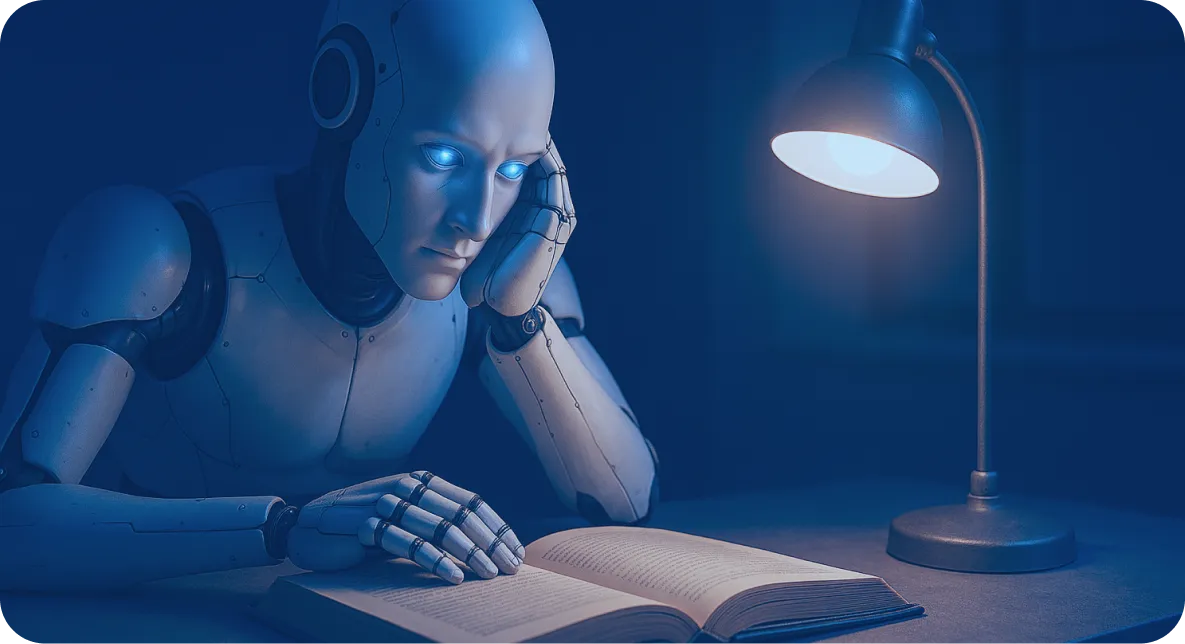
Key Highlights
- Self-learning AI adapts and improves autonomously using unlabeled data, boosting AI learning and decision-making
- Supervised, unsupervised, and reinforcement learning allow AI to continuously optimize its performance in any industry
- Self-learning AI reduces reliance on human supervision, offering faster adaptation, real-time analytics, and increased efficiency with limited data
What Is Self-Learning AI?
Self-learning AI represents a type of artificial intelligence system that independently trains itself using machine learning algorithms unlabeled data to identify patterns and draw conclusions. Unlike traditional systems that rely on labeled data, this AI system mimics the concept of immersive learning, allowing it to adapt and improve without explicit programming. By leveraging a neural network and machine learning models, self-learning models accelerate the learning process and augment AI learning in all industries.
Types of Self-Learning AI
Self-learning systems based on AI continuously improve by analyzing data and adapting without explicit human supervision. Unlike explicitly programmed systems, self-learning models evolve by recognizing patterns in data and building their AI skills over time without requiring manual intervention. Let’s review the different types of self-learning AI.

Supervised Learning AI
Supervised learning AI uses labeled data to train models, where each input is associated with a specific output. This process allows the system to learn patterns and make accurate predictions on new data. The model adjusts based on labeled examples to reduce prediction errors, effectively building parametrically logical systems that can be applied to tasks like classification and regression.
Unsupervised Learning AI
Unsupervised learning AI works with unlabeled data, allowing the model to find patterns or structures independently. It is used for clustering data into groups or discovering associations between variables. Without predefined labels, these systems autonomously uncover insights, such as customer segments or anomalies, making it ideal for large, unstructured datasets.
Reinforcement Learning AI
Reinforcement learning AI uses trial and error to learn optimal behaviors for a specific environment. The system receives rewards for correct actions and penalties for wrong ones, guiding its decision-making process. Over time, AI refines its strategy to maximize rewards, demonstrating continuous model training and improvement in tasks that require ongoing adaptation, like robotics, gaming, and autonomous systems.
Benefits of Self-Learning AI
Self-learning AI systems utilize advanced AI technology like artificial neural network hardware and natural language processing to accelerate learning and decision-making. Let’s examine several key benefits of self-learning artificial intelligence.
Faster Learning

Self-learning AI accelerates adaptation by analyzing input data without relying on labeled data, speeding up development compared to traditional methods. This benefit reduces the reliance on manual training machines and provides a solid understanding of how AI can perform tasks in various contexts.
Efficiency with Limited Data
Self-learning AI excels in scenarios with minimal training data, deriving insights from incomplete datasets and recognizing patterns that would typically require large amounts of labeled data. This ability makes it ideal for self-learning systems, where the system can adapt and improve without extensive formal learning or additional training processes.
Skill Transferability
Once trained, self-learning AI transfers newly acquired skills to different disciplines, improving versatility and adaptability for almost any application. This flexibility boosts the learning model as it is influenced by real-world scenarios, allowing it to handle new challenges and perform tasks in multiple settings.
Real-Time Analytics
Self-learning systems utilize real-time decision-making by analyzing data as it enters the system, promoting accuracy and reducing response times for dynamic environments. Accurate real-time analytics can mean the difference between success and failure for many companies.
Reduced Human Supervision
These systems minimize reliance on human researchers, reducing the need for manual dataset creation, which expedites AI deployment and the training of machines. This level of independence makes self-learning systems an increasingly important aspect of artificial intelligence.
Improved Robustness
Self-supervised learning strengthens the ability to handle a range of challenges and reduces training costs, making them more effective in real-world situations. By using techniques such as inverse reinforcement learning, these systems can adapt to complex, dynamic environments.
Examples of Self-Learning AI
Self-learning AI and natural language processing (NLP) are making significant strides in several industries, including cybersecurity, healthcare, and finance. Some prime examples of self-learning AI include:
- Cybersecurity — Self-learning systems continuously adapt to new threats, improving detection speed and system security. The AI cybersecurity system identifies anomalies using unsupervised learning and training processes, reducing the need for constant updates and intervention by human researchers.
- Healthcare — AI models analyze large amounts of labeled data and patient information, using neural networks to identify early signs of diseases. By spotting patterns that human researchers might miss, AI systems suggest personalized treatments, improving healthcare outcomes.

- Finance — Self-learning AI systems in finance detect fraudulent activities by learning from past transactions. They flag anomalies and suspicious activities using training processes to minimize financial risks, providing faster responses than traditional systems.
- Retail — Self-learning systems continuously adapt to customer interactions, bolstering applications like chatbots. These AI retail systems improve their AI skills through deep learning, refining responses and accuracy over time.
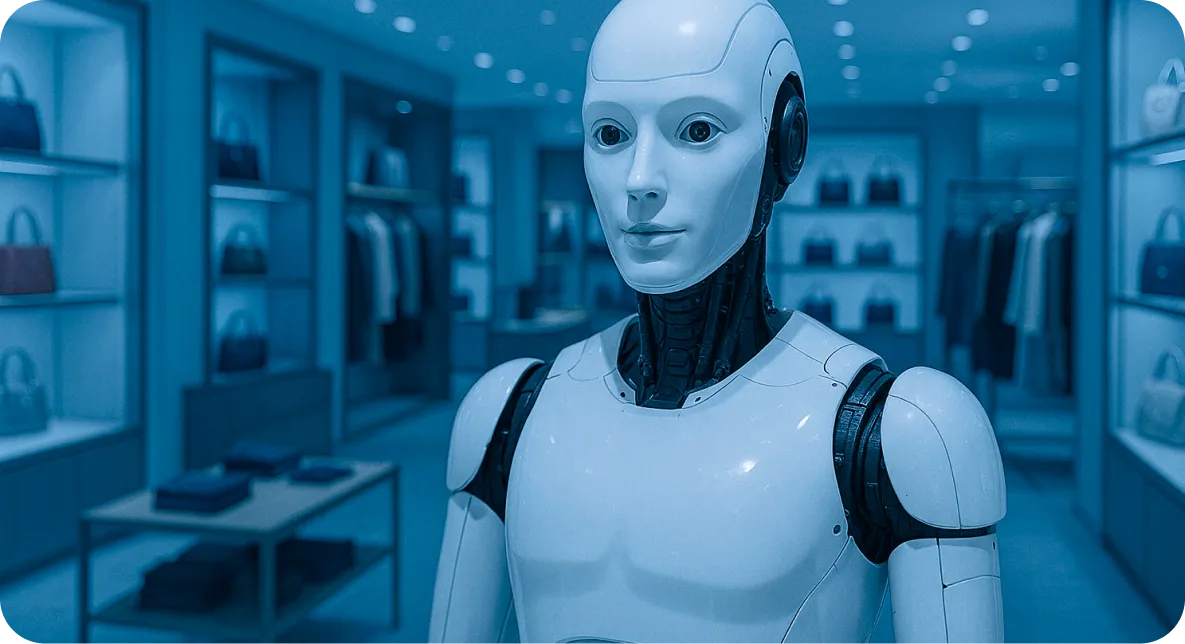
How Does Self-Learning AI Work?
Self-learning AI adapts and improves through continuous interaction with data environments. But how does self-learning AI function?
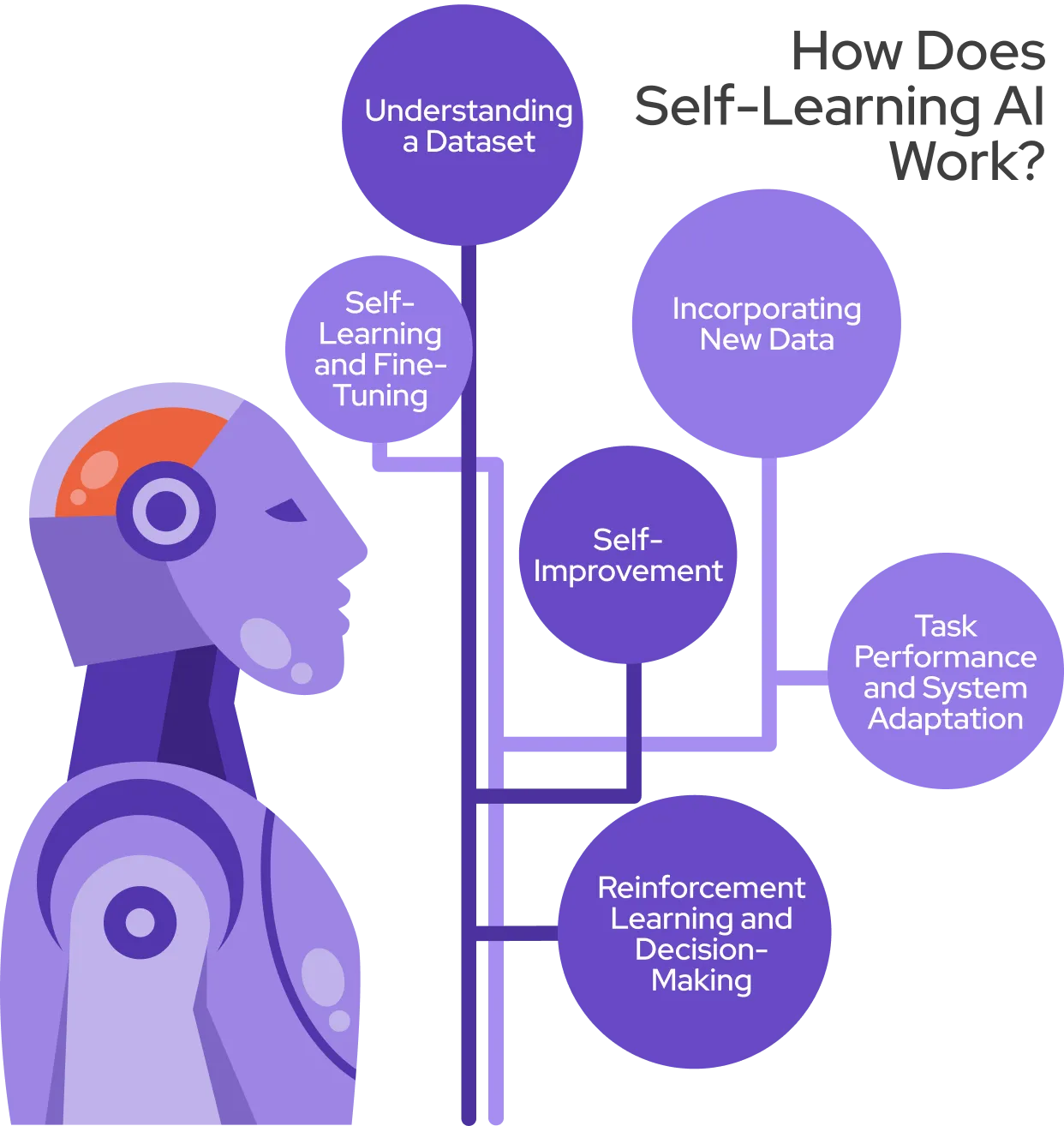
It works by:
- Understanding a Dataset — Once the unlabeled data is processed, the system autonomously identifies patterns and relationships, much like how humans learn by observing and interpreting their environment without predefined labels.
- Self-Learning and Fine-Tuning — Through self-supervised learning, AI systems fine-tune their performance by improving with each interaction and adjusting their learning models based on real-time feedback.
- Incorporating New Data — Unlike traditional systems, a self-learning system autonomously integrates new data without retraining from scratch, allowing the system to optimize its performance dynamically.
- Task Performance and System Adaptation — AI models process complex data to complete tasks efficiently. These systems use software structures to amplify performance, adapt to new conditions, and improve based on training processes.
- Self-Improvement — The learning model continuously refines its skills, responding to changing environments. This plasticity improves decision-making and strengthens human intelligence capabilities when used as a tool.
- Reinforcement Learning and Decision-Making — Self-learning systems optimize decision-making through trial-and-error methods, such as inverse reinforcement learning. By evaluating actions based on rewards and penalties, the system fine-tunes its behavior to maximize rewards, improving its effectiveness.
Self-Learning AI Use Cases
A self-learning system has revolutionized multiple industries by enabling machines to adapt autonomously. Several common applications for self-learning AI have emerged in recent years. Key use cases include:
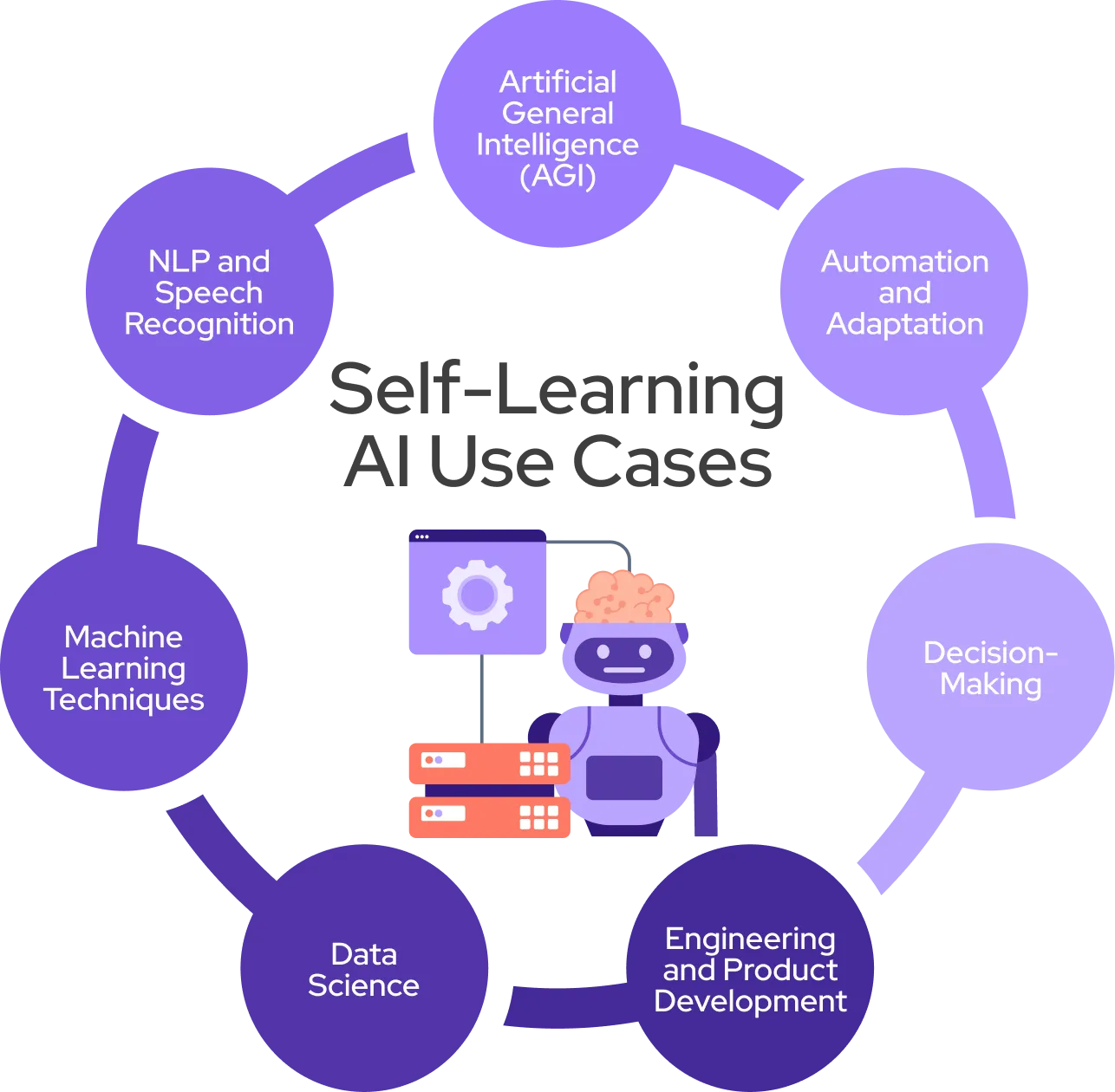
- Artificial General Intelligence (AGI) — Self-learning AI could potentially evolve into AGI, where systems learn almost everything about most industries, building their AI skills and expanding the breadth of tasks they can perform autonomously.
- Automation and Adaptation — These systems offer scalable solutions, allowing self-learning systems to improve without explicit programming. Their ability to adapt and optimize in real time makes them ideal for industries needing automated decision-making.
- Decision-Making — Self-learning models augment decision-making, especially in dynamic environments like racing, where the AI adjusts in real time to improve performance based on data analysis.
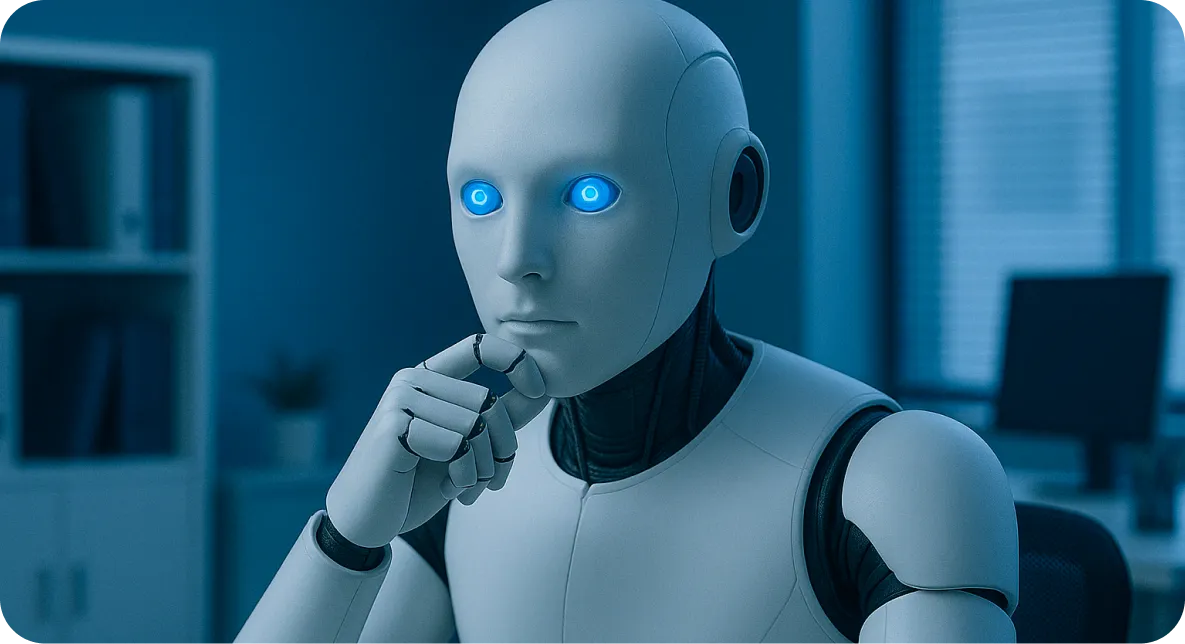
- Engineering and Product Development — AI is optimizing product testing and fault detection by reducing reliance on extensive physical testing and leveraging machine learning methods to speed up development.
- Data Science — A self-learning system accelerates model development by reducing dependence on labeled data, improving AI learning for data scientists who need faster and more efficient solutions.
- Machine Learning Techniques — A self-learning model developed by a machine learning engineer tackles complex data challenges and adapts to evolving tasks. These machine learning models mirror human learning, continuously improving by interacting with the data environment and refining their performance through an ongoing training process.
- NLP and Speech Recognition — Self-learning models adapt by processing labeled data and refining their capabilities over time. This evolution allows them to better understand and respond to natural language, showcasing the key advantages of artificial narrow intelligence where the system excels in specific tasks without broader cognitive capabilities.
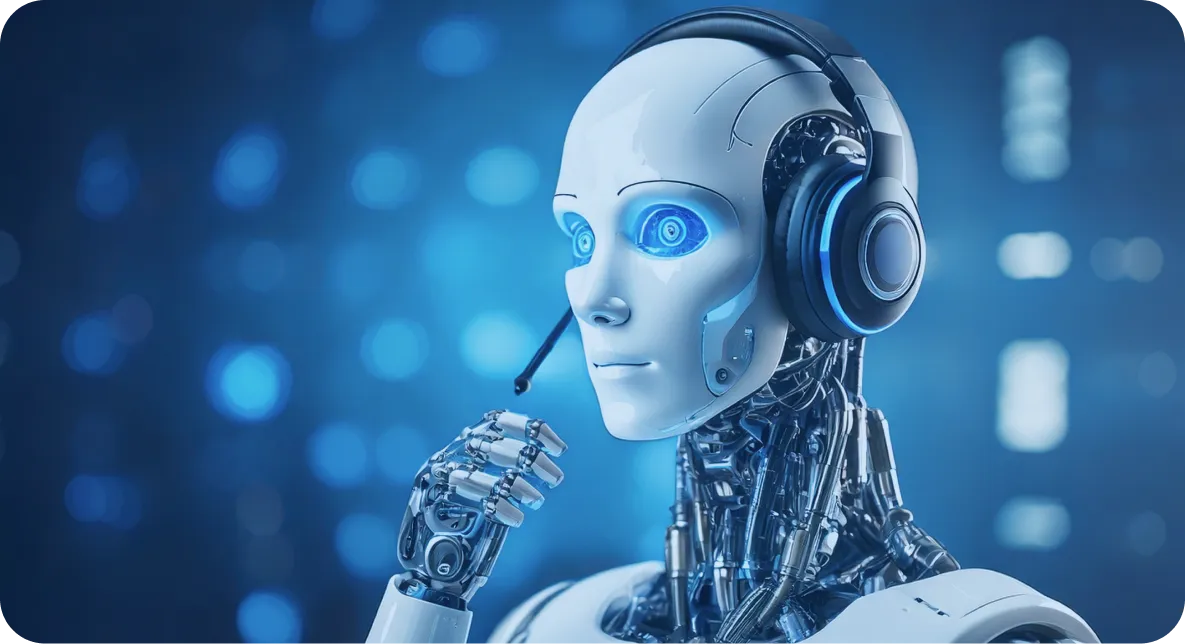
Artificial Intelligence, Self-Learning, and More | AIFA Labs
Ready to let self-learning AI work for your company? The Cerebro Generative AI Platform offers a full suite of cutting-edge artificial intelligence tools for business. Store and retrieve company data with Cerebro Knowledge AI. Produce low-code/no-code AI apps with Cerebro Flow AI. And manage your employees’ AI usage with the Cerebro AI Governance and Observability Platform (AGOP). Book a free demo online or call AiFA Labs at (469) 864-6370.