Find out what enterprise AI can do for your business! As artificial intelligence (AI) continues to sweep through most industries, more companies are investigating how to implement it. AI offers many benefits and presents a few new challenges. Discover examples of how the top enterprises use AI below.
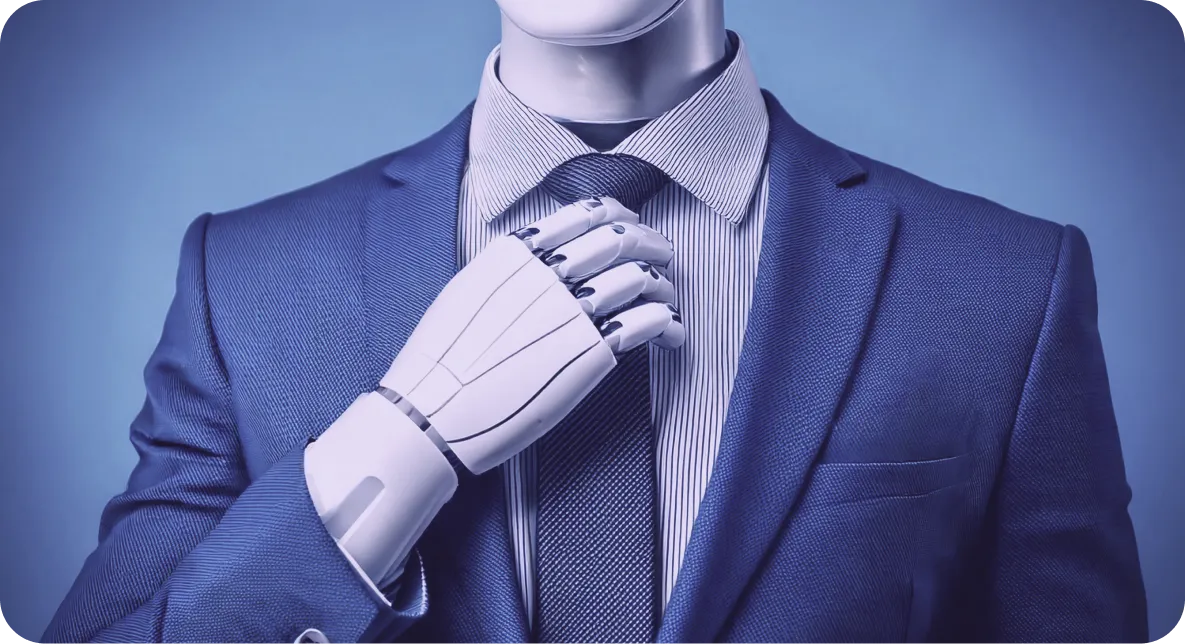
What Is Enterprise AI?
Enterprise AI is artificial intelligence technology that solves business problems and improves operations for large organizations. It includes infrastructure, policies, and strategies for company-wide use. Artificial intelligence in enterprises leverages machine learning (ML), deep learning, and natural language processing (NLP) to increase efficiency, resilience, profitability, and eco-friendliness.
What Is an Enterprise AI Platform?
An enterprise AI platform is a framework that streamlines the development, deployment, and scaling of AI solutions. It integrates data, experimentation, operations, and user experience technologies, promoting collaboration between AI specialists and engineers. Business AI software drives scalability, efficiency, and continuous improvement through deep learning models.
Examples of How AI Is Being Used in Enterprises

AI and machine learning stand at the forefront of operational transformation for enterprises. Managing AI models improves how businesses operate by optimizing business processes and adapting to evolving market demands. Best practices for the latest AI techniques in various business applications include:
- Application Development — Large language models automate code generation, speeding up software development and allowing developers to focus on higher-level tasks
- Customer Operations — AI-embedded systems personalize customer interactions, improving response times and freeing up human agents for more complex issues
- Marketing — AI customizes marketing campaigns using customer data, boosting conversions and improving customer engagement
- Sales — AI virtual sales reps guide prospects with personalized interactions, boosting sales efficiency
- Risk and Legal — AI automates contract creation, maintains compliance, and translates documents for global operations
- Maintenance — Predictive maintenance AI monitors equipment performance to prevent failures and reduce downtime
- Decision-Making — AI analytics support informed, real-time decision-making, improving business strategy
- Chatbots — AI chatbots and virtual assistants efficiently manage routine inquiries, streamlining customer service and internal operations
- Data Protection — AI ensures compliance with data protection regulations and automates privacy request management
- Fraud Detection — AI detects fraudulent transactions in real-time, improving security and increasing trust
Benefits of Enterprise AI
AI offers organizations many benefits that improve efficiency, drive innovation, and support strategic growth. By incorporating AI into business operations, companies unlock new capabilities and refine processes. Find some of the main benefits of enterprise AI below.

Increased Efficiency and Productivity
By automating repetitive tasks, streamlining workflows, and optimizing resource allocation, AI allows employees to focus on core duties. As a result, organizational processes accelerate, timelines shrink, and organizations experience an immediate return on investment.
Improved Customer Experience
The latest AI-powered chatbots, virtual assistants, and personalized recommendation systems engage customers in more meaningful, responsive ways. By anticipating customer needs, personalizing interactions, and providing 24-hour support, AI increases customer satisfaction and loyalty.
Cost Reduction
Through business process optimization and task automation, business AI significantly reduces costs. By eliminating inefficiencies, improving resource utilization, and streamlining workflows, AI solutions result in better financial outcomes and sustainable growth.
Inspired Innovation
By democratizing access to cutting-edge technologies in all departments, enterprise AI allows teams to experiment with and integrate the latest AI techniques into their operations. Consequently, it fosters a culture of innovation so businesses can stay competitive and adapt to shifting markets.
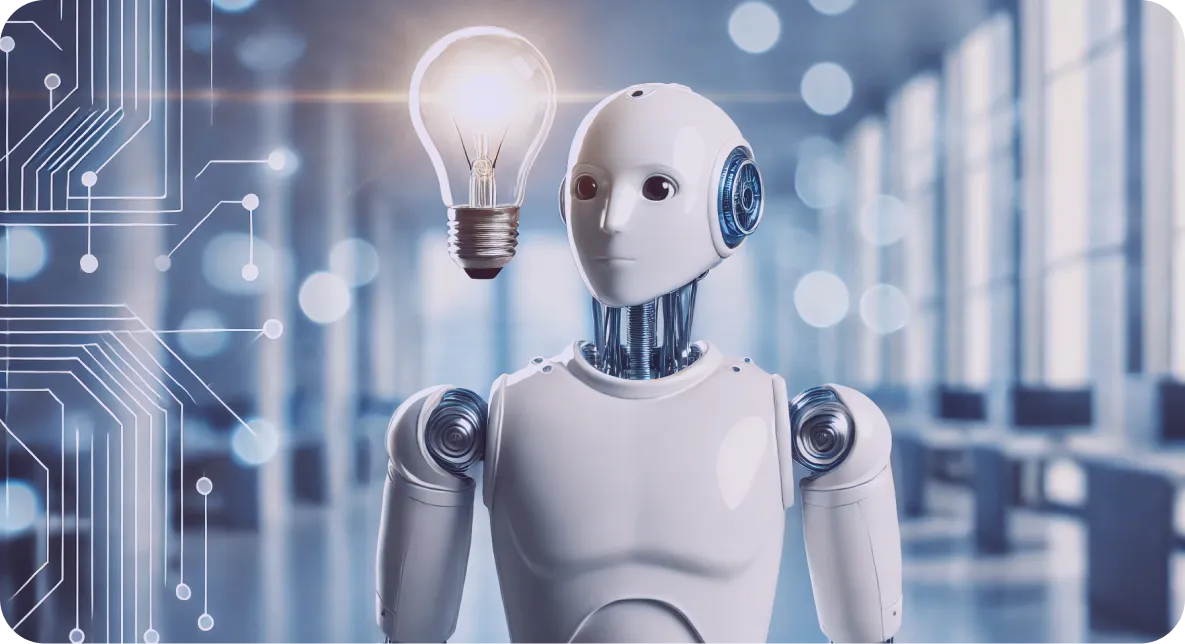
Informed Decision-Making
AI tools provide actionable, data-driven insights that improve forecasting accuracy and support scenario analysis. By analyzing large datasets in real-time, AI empowers businesses to make informed, timely decisions, aligning strategies with evolving market conditions.
Better Governance and Transparency
Enterprise AI platforms offer robust controls over sensitive data. Explainable AI allows businesses to trust AI-powered decisions, maintain regulatory compliance, and promote transparency. As a result, it mitigates risks and builds confidence among stakeholders.
Competitive Advantage
Leveraging AI to refine business operations and accelerate innovation provides companies with a competitive edge. AI equips organizations with the tools to adapt to customer demands, adjust product offerings, and identify new business opportunities, strengthening their market positions.
Workforce Improvements
AI improves the workforce by augmenting employee capabilities. Tools like productivity assistants, adaptive learning platforms, and workforce analytics boost employee performance and satisfaction, creating a more efficient, engaging work culture.
Challenges of Enterprise AI
Organizations must navigate concerns related to privacy, security, and ethics, all of which unlock the full potential of AI while maintaining trust and compliance. Let’s explore some of the enterprise AI challenges organizations face during implementation.
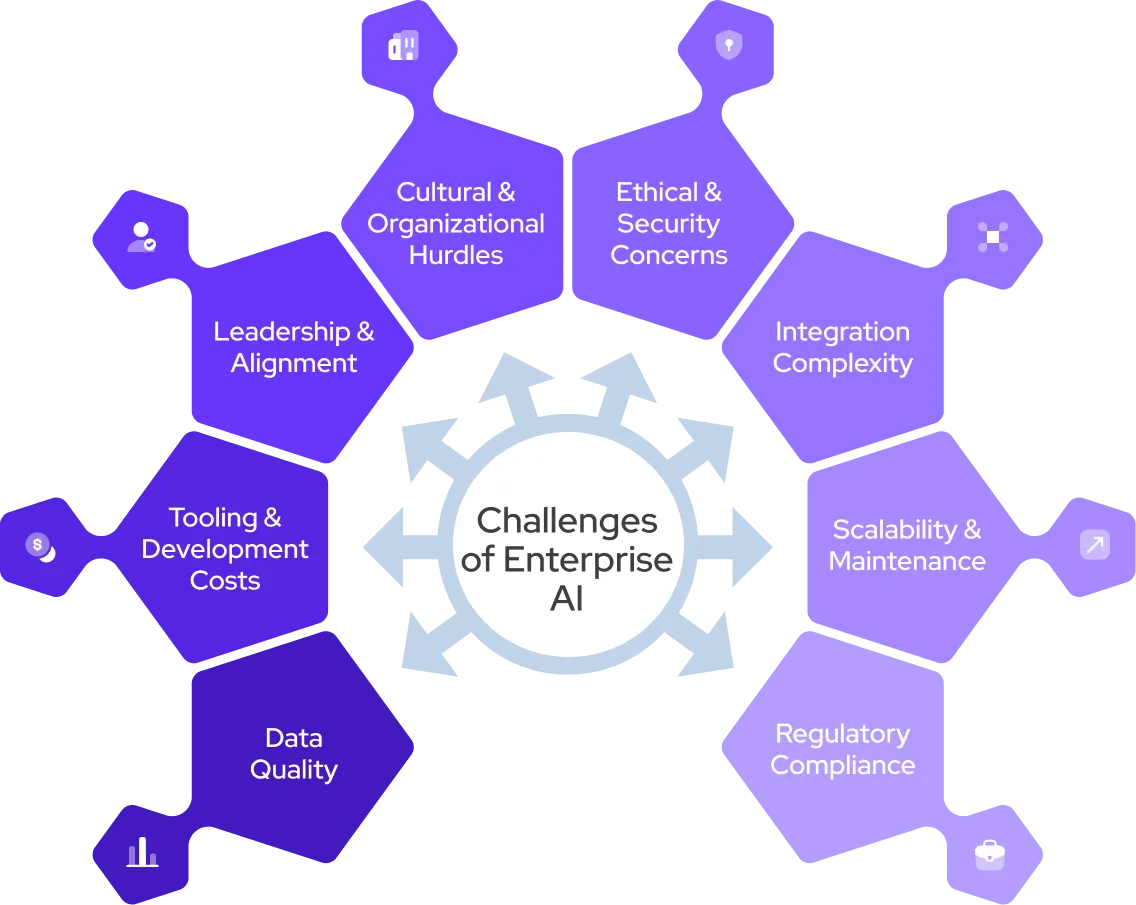
Data Quality
AI systems rely on high-quality data. Irrelevant, biased, or poor-quality data leads to inaccurate models. Achieving optimal AI performance requires that the data remain relevant, unbiased, and secure.
Tooling and Development Costs
Developing AI systems in-house proves expensive and demands specialized expertise and tools. Smaller organizations often turn to outsourcing, but attaining seamless compatibility with existing systems also presents significant challenges.
Leadership and Alignment
Clear leadership is essential for all AI projects. In large organizations, overlapping roles and competing opinions hinder progress. A unified vision and strong leadership lead to successful AI adoption.
Cultural and Organizational Hurdles
AI may face resistance from employees concerned about job displacement or work disruption. Transparent communication, training, and support are necessary to foster acceptance and confidence in AI tools.
Ethical and Security Concerns
AI introduces ethical challenges such as bias, fairness, and data privacy. Businesses must implement strict ethical guidelines and security measures for responsible AI use and data protection.
Integration Complexity
Integrating AI with legacy systems can become complex and disruptive. A phased approach, starting with pilot projects, helps identify integration challenges early and facilitates smooth adoption.
Scalability and Maintenance
Scaling AI solutions and maintaining their performance over time requires ongoing investment in infrastructure and resources. Continuous monitoring and updates keep AI systems effective and aligned with business needs.
Regulatory Compliance
AI systems must comply with evolving regulations concerning data privacy and security. Staying informed about compliance requirements reduces potential legal and financial risks.
Technological Considerations for Enterprise AI
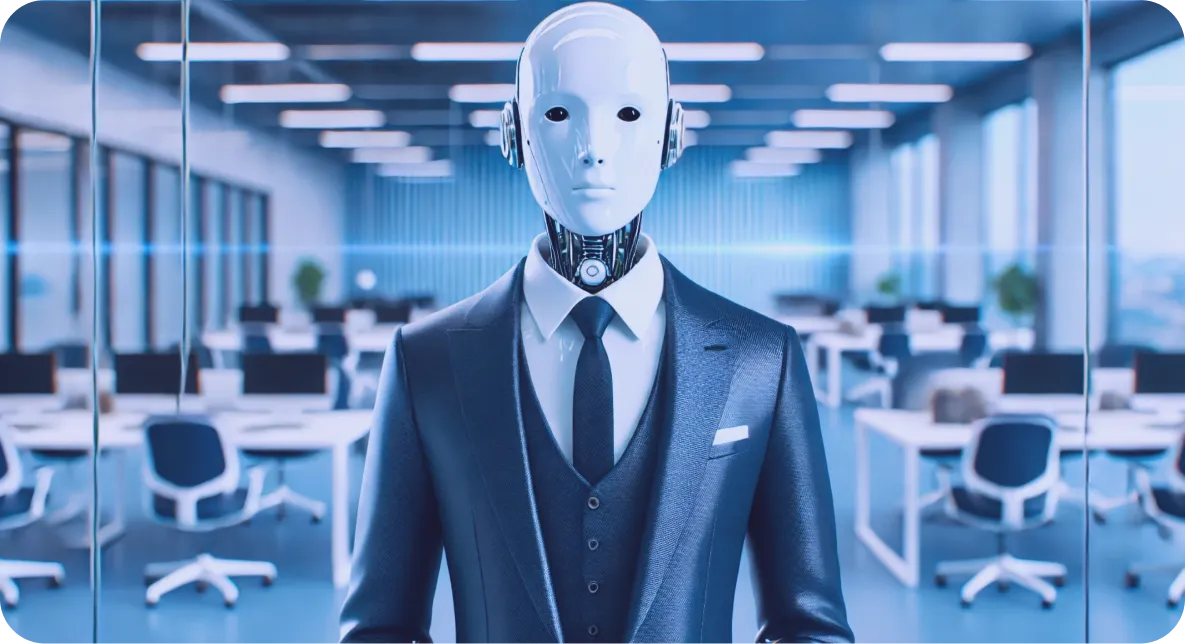
Businesses intending to deploy AI must address the fundamental technological factors that drive scalability, security, and operational efficiency. These considerations are vital for achieving successful integration and long-term value. Some of the most important technological factors to consider when adopting enterprise AI include:
- Advanced Analytics and Automation — AI-powered analytics and automation streamline processes, provide predictive insights, and free up resources for strategic tasks
- Central Model Registry — A model registry tracks AI model versions, supports version control, and maintains metadata for collaboration, compliance, and data governance among teams
- Collaboration and User Experience — User-friendly interfaces and collaborative tools support seamless interaction with AI systems, fostering organization-wide adoption and efficient decision-making
- Data Management — Robust data pipelines and centralized governance provide secure, efficient access to enterprise data. Tools like data catalogs assist with quick data discovery while maintaining compliance
- Integration — Seamless integration with existing IT systems, like ERPs and CRMs, is vital for streamlining workflows and driving enterprise-wide objectives
- Model Deployment — MLOps and LLMOps streamline the AI development lifecycle by automating stages like training, testing, and deployment, using CI/CD pipelines to enable rapid iteration and real-time updates
- Model Monitoring — Continuous monitoring assures AI model reliability and relevance, using human-in-the-loop (HITL) mechanisms and real-time feedback to maintain accuracy
- Model Training Infrastructure — A centralized infrastructure for training machine learning models, such as feature stores and retrieval-augmented generation (RAG), enables collaboration and prevents duplicate efforts
- Reliability and Availability — High availability, redundancy, and disaster recovery plans preserve business continuity and minimize downtime
- Scalability — AI systems must be able to scale horizontally and vertically, optimizing resource management to handle large, complex datasets and growing demands
- Security and Compliance — Robust security measures and adherence to regulatory frameworks, such as GDPR and HIPAA, promote data protection and reduce risk
How to Implement Enterprise AI in 10 Steps
AI projects rely on clear objectives, robust data infrastructure, and cross-functional collaboration. Following AI best practices, such as close monitoring, model optimization, and maintaining scalability, remains crucial for long-term success. Implementing enterprise AI requires a strategic, well-structured approach. Here are 10 crucial steps to facilitate effective deployment.
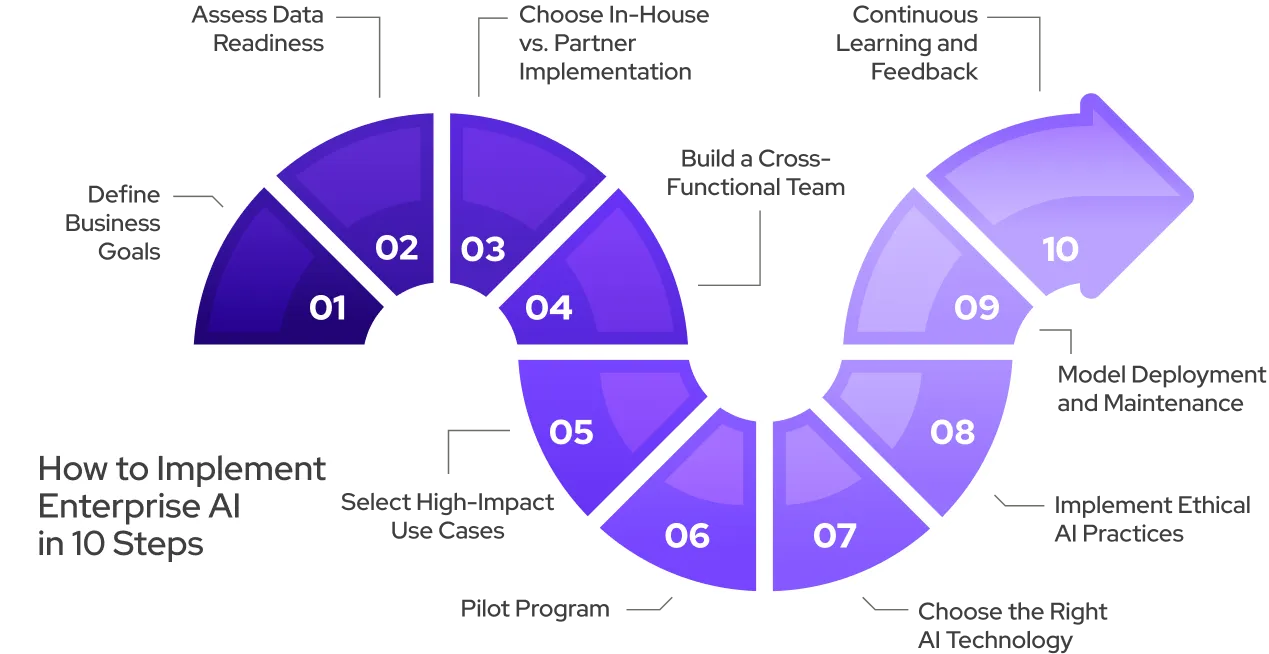
Step 1: Define Business Goals
Clearly outline the problems AI will solve and the opportunities it will seize. Establish measurable objectives aligned with overall business strategies to focus efforts on impactful outcomes.
Step 2: Assess Data Readiness
Evaluate the quality, volume, and accessibility of your data. Identify gaps, and implement a clean, organized, and compliant data strategy with strong governance for security and privacy.
Step 3: Choose In-House vs. Partner Implementation
Decide whether to implement AI using in-house resources or by partnering with an expert provider. In-house offers control but requires significant resources and expertise. Partner-led implementation accelerates the process but comes at a higher cost.
Step 4: Build a Cross-Functional Team
Form a team of data scientists, engineers, IT specialists, and domain experts to collaborate with various departments. This multidisciplinary approach will confirm AI solutions are both technically robust and aligned with business needs.
Step 5: Select High-Impact Use Cases
Identify AI use cases that align with business objectives and provide significant value. Prioritize use cases that appear feasible with available data and offer clear ROI.
Step 6: Pilot Program
Launch a pilot program to test the AI solution on a small scale. Start with a specific use case, gather feedback, and make adjustments based on insights before scaling up. This AI strategy reduces risks and promotes a smoother full-scale deployment.
Step 7: Choose the Right AI Technology
Select AI-powered technologies tailored to your specific requirements, facilitating scalability and alignment with your business objectives. Consider factors like performance, compatibility, and ease of integration with existing systems.
Step 8: Implement Ethical AI Practices
Develop ethical guidelines around fairness, accountability, and transparency in AI systems. Regularly audit models to identify and mitigate biases, safeguarding ethical alignment in all AI-powered decisions.
Step 9: Model Deployment and Maintenance
Use MLOps to automate the AI lifecycle, including training, testing, and deployment. Monitor model performance and adapt to evolving data and business needs through periodic updates and retraining.
Step 10: Continuous Learning and Feedback
Establish feedback loops and ongoing training programs to keep employees informed and adaptable as AI technologies evolve. Assess the AI system’s effectiveness in order for it to remain aligned with business goals and user needs.
What Is the Difference Between Regular AI and Enterprise AI?
While both regular artificial intelligence and enterprise AI utilize artificial intelligence technologies, they serve different purposes and operate at varying scales. Standard AI focuses on specific tasks, whereas enterprise AI addresses complex, large-scale challenges within an entire organization. Here’s a breakdown of the differences between the two.

Collaboration and Usability
- Regular AI: Primarily designed for individual use, with limited collaboration functionalities
- Enterprise AI: Facilitates collaboration with multiple departments and stakeholders within an organization, integrating AI into business workflows for broader organizational impact
Customization and Flexibility
- Regular AI: More generic, often providing off-the-shelf solutions with limited customization
- Enterprise AI: Highly customizable to meet specific organizational needs, allowing tailored solutions for various complex business processes
Data Integration
- Regular AI: Operates on a narrow dataset, focused on personal data like voice recordings or browsing history
- Enterprise AI: Integrates data from multiple sources, including sensitive business information and proprietary data, to provide comprehensive insights
Scope and Scale
- Regular AI: Focuses on individual tasks or personal applications, such as virtual assistants (e.g., Siri, Alexa) and recommendation systems; handles limited, personal data for single-user interactions
- Enterprise AI: Designed for large-scale, complex enterprise environments, business AI integrates into various processes, handling massive datasets and sophisticated analytics to boost productivity
Security and Compliance
- Regular AI: Generally less focused on advanced security measures because it deals with personal data in non-sensitive contexts
- Enterprise AI: Includes robust security features to safeguard sensitive business and customer data, maintaining compliance with regulatory requirements such as GDPR or HIPAA
Types of Enterprise Artificial Intelligence
Enterprise AI encompasses various technologies that meet complex business challenges, automate tasks, and fine-tune operations. AI solutions provide targeted strategies for optimizing business operations, as outlined below.
Enterprise AI Use Cases by Industry
An effective enterprise AI strategy brings significant improvements to businesses by increasing customer satisfaction and addressing complex industry challenges. Below, find several use cases for enterprise AI that demonstrate its transformative potential within multiple sectors. These applications foster innovation and position organizations for sustainable growth and competitive advantage.
Automotive
An enterprise AI platform propels manufacturing efficiency and safety by leveraging computer vision for defect detection and predictive maintenance. Autonomous driving technologies use deep learning for real-time sensor data processing, bolstering navigation and safety.
Energy
Enterprise AI solutions maximize energy efficiency and integrate renewable sources by predicting demand and reducing waste. Smart grids powered by AI promote sustainable, cost-effective energy management.
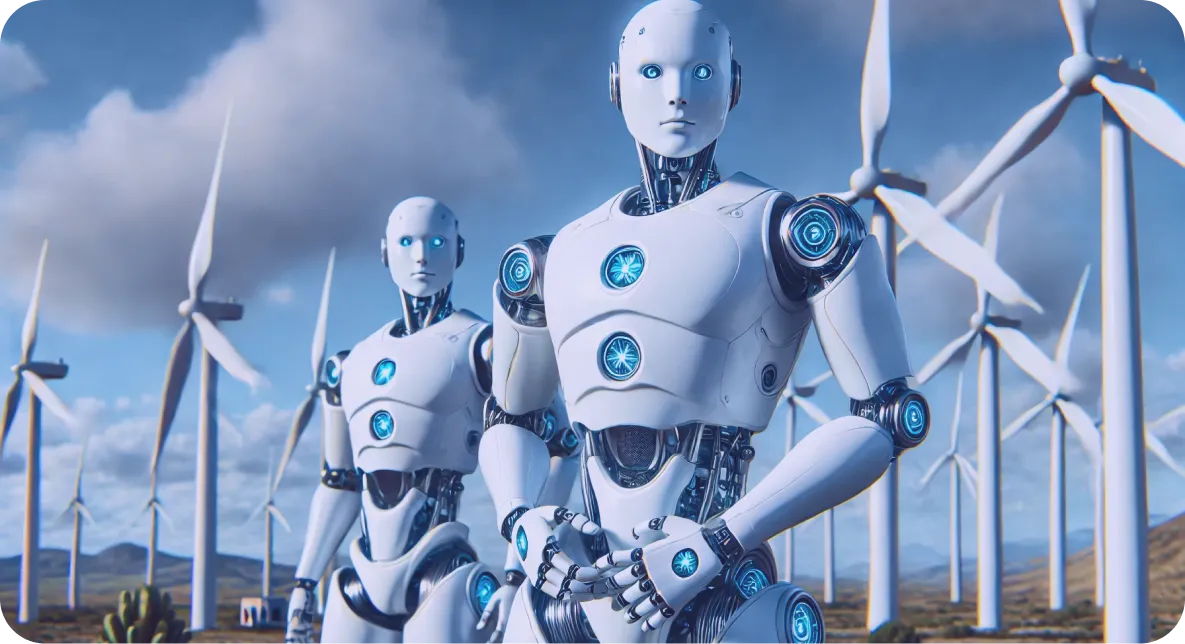
Entertainment
AI applications augment the user experience through personalized recommendations and dynamic game environments. It also accelerates content creation and automates post-production, reducing costs and improving productivity.
Finance
AI projects boost security with fraud detection, provide personalized investment strategies via robo-advisors, and optimize risk management. Business AI supports data-driven decision-making for financial institutions.
Healthcare
An AI platform increases diagnostic accuracy by analyzing medical images and detecting diseases early. It also streamlines administrative tasks, improving operational efficiency and freeing up resources for patient care.
Life Sciences
Enterprise software accelerates drug discovery and genomic research, developing personalized treatments and reducing the time and cost spent on research, leading to faster market availability.
Manufacturing
An AI platform in manufacturing facilitates predictive maintenance, reduces downtime, and optimizes supply chains. It also elevates quality control, improving product quality, safety, and efficiency.
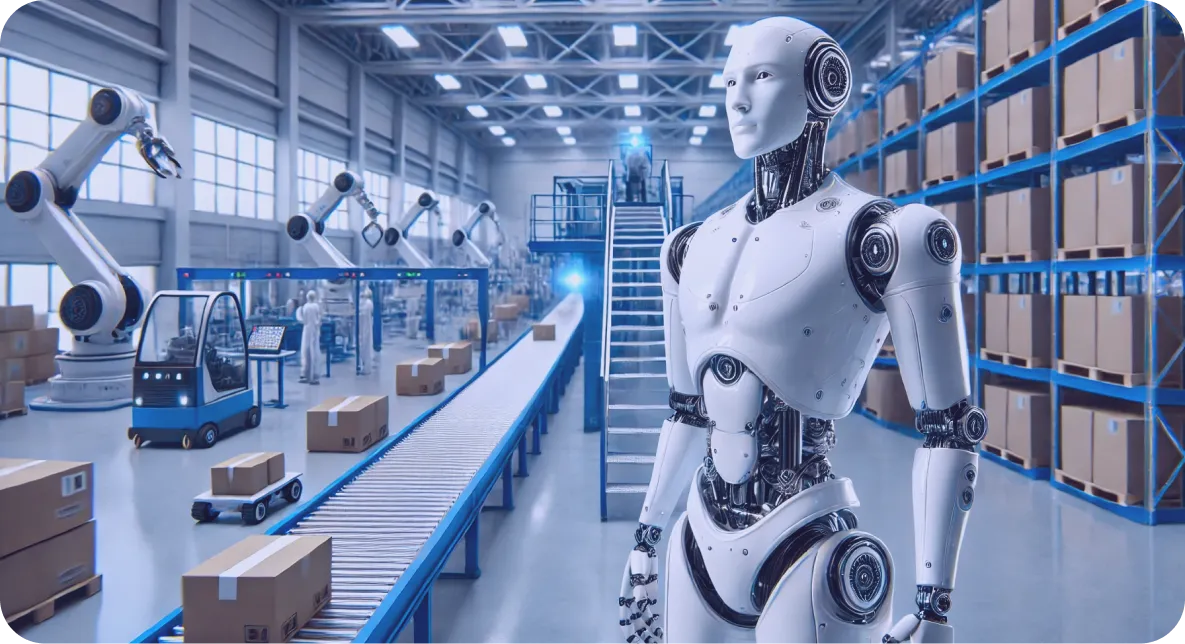
Public Sector
AI applications improve government services and citizen engagement through chatbots and predictive analytics, supporting urban infrastructure management, public safety, and data-driven policy-making.
Software Development
AI tools accelerate software development by automating code generation, testing, and security checks, leading to faster delivery of secure, high-quality software.
Telecom
Enterprise AI solutions optimize network performance, predict issues, and boost customer service through personalized experiences, elevating customer satisfaction while reducing costs.
By embedding enterprise AI applications into core business operations, companies can streamline workflows and unlock a significant competitive advantage. When businesses analyze sensitive data, identify patterns, and make more informed decisions, it positions them to thrive in an AI-powered global economy.
AiFA Labs | Your Enterprise AI Company
Modernize your business with one of the best enterprise AI solutions on the market: the Cerebro Generative AI Platform. Cerebro consists of 15 distinct AI products in one program, including Vision AI, SAP AI SDLC Assist, Safety AI, and more. Book a free demonstration online or call AiFA Labs at (469) 864-6370 today.